Advanced industrial analytics helps engineers predict when maintenance is needed and provide prescriptive instructions.
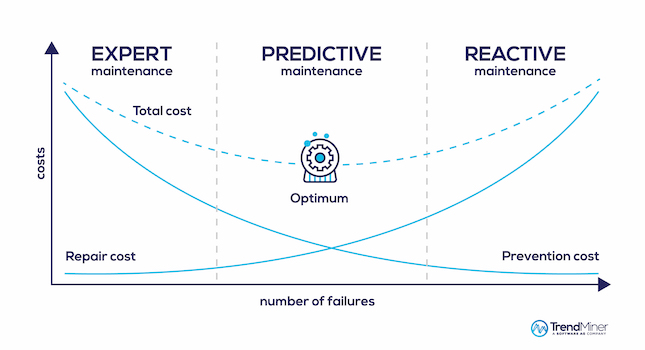
Industrial analytics insights
- Predictive maintenance focuses on forecasting equipment behavior to schedule maintenance, while prescriptive maintenance goes further by using analytics to provide outcome-based recommendations for maintenance actions.
- Operational experts play a crucial role in determining the right time for maintenance, as they possess the necessary knowledge to differentiate between good and bad process behavior and failure modes.
- Advanced industrial analytics, collaboration between engineers and data scientists, and the integration of contextualized data enable engineers to achieve the benefits of predictive and prescriptive maintenance, optimizing maintenance schedules and improving operational efficiency.
Since the emergence of Industry 4.0, the process manufacturing industry has paid considerable attention to predictive operational performance. This discussion revolves around predictive maintenance. Engineers can leverage sensor-generated data to forecast equipment behavior and use this information to schedule the right time to perform maintenance on critical assets.
Prescriptive maintenance, however, is more than that. Rather than determining when equipment might fail, the goal of prescriptive maintenance is creating outcome-based recommendations through prescriptive analytics. Operational experts then can use these recommendations to provide instructions to the team performing the maintenance.
Predictive and prescriptive maintenance require the knowledge of a process expert. This includes the ability to tell the difference between good and bad process behavior and related failure modes. Therefore, determining the right time to perform maintenance is a job best left to operational experts.
How can engineers leverage operational data to achieve the benefits of predictive maintenance and provide prescriptive measures? What solutions can they apply to gain insights on the data? And how do engineers and data scientists collaborate for even greater improvements?
From predictive to prescriptive maintenance
Predictive maintenance can be defined as the effort to reach a high level of asset reliability while reducing unnecessary maintenance costs by decreasing the maintenance frequency. Good predictive maintenance practice ensures maintenance work is planned at the right time before an asset fails or performance becomes degraded.
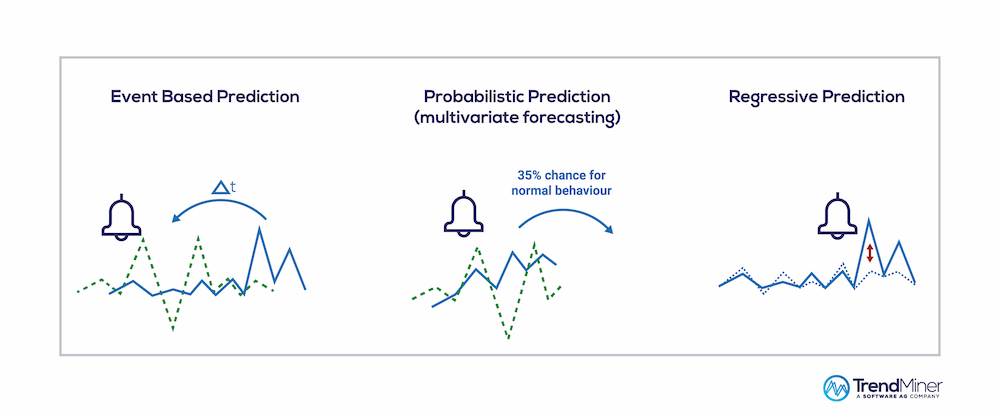
Similar approaches to predictive maintenance differ in complexity but have the same goal. These include failure finding maintenance (FFM), risk-based maintenance (RBM) and condition-based maintenance (CBM). Although some consider predictive maintenance to be an advanced form of CBM, it often uses data from more sources and can detect when operating zones are shifting. Predictive maintenance also automatically detects patterns and sends alerts earlier than a system using simple logic rules.
On the other hand, prescriptive maintenance is an advanced maintenance strategy that combines analytics, condition monitoring sensors and machine learning to predict equipment failures and recommend specific actions to prevent them. Prescriptions could be generated for either predictive or preventative maintenance approaches and therefore an engineer’s reasoning skills are required. Prescriptive maintenance also needs even more data. To create an adequate prescription, engineers must use historical information to assess all possible failure circumstances.
Creating a data-driven prediction
As shown in figure 1, advanced industrial analytics uses three different prediction approaches. The first is based on events. For example, engineers can receive an alert with instructions if an event occurs that could negatively affect another part of the process. The second approach is probabilistic. In this approach, advanced industrial analytics reviews the current process behavior, determines if a failure is likely and automatically schedules work orders with instructions if necessary. The third type of approach is regressive, which requires certain conditions be met and verified before maintenance receives work orders or instructions.
With advanced industrial analytics, occurring events are captured for use in future predictions and prescriptions. Adding information from third-party sources puts events in their operational context. This includes data from sources such as maintenance systems, laboratory information systems, overall equipment efficiency (OEE) systems, batch systems or even weather reports.
Collaborating with central data teams on a machine learning exercise provides even more opportunities for stronger predictions. Engineers can use captured events and other unprocessed, pre-processed and processed data to develop an anomaly detection model or failure analysis.
Applying a prescriptive approach
Predictive maintenance aims to schedule maintenance at a time not only when it is the most cost-effective, but also when it will have the least effect on operations (see Figure 2). To get the prescription for each possible prediction or to learn the best time for preventive maintenance, all equipment and process-related sensor data should be used. This is called “contextualization of asset performance with process data,” which makes data-driven prescriptive maintenance become possible.
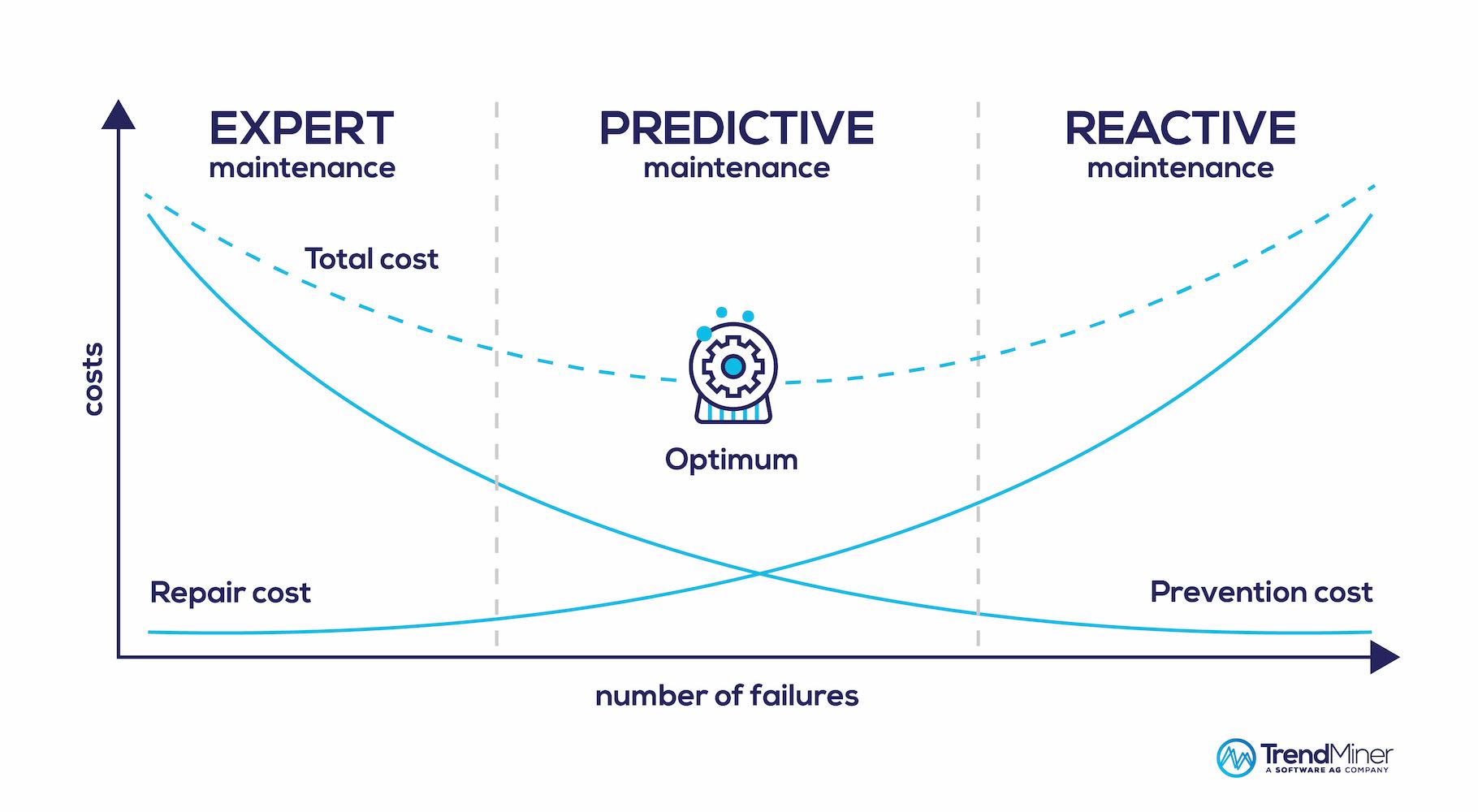
One example is the fouling of heat exchangers. Fouling increases cooling time and slows down production. When a heat exchanger is used for different product grades and recipes, it becomes almost impossible to monitor fouling. Scheduling maintenance too early leads to unwarranted downtime, but performing maintenance too late leads to degraded performance, increased energy consumption and potential safety risks. Using advanced industrial analytics, engineers set up a monitor to look at the cooling times of their most recent products. If the duration of the cooling phase starts to increase, a warning is sent to the engineers who then provide maintenance with instructions on cleaning the reactor.
In another case, a breakthrough filter used for removing impurities also suffered from fouling. This happens in batches when temperature and pressure increase slightly. Sometimes, one of the valves leaks, which causes gas and debris to enter the system. The valve then sticks from solid buildup until the filter eventually breaks. For this process, operational experts established a monitor that watched for leaking valves. This let them know the breakthrough filter was about to break so they could schedule maintenance and provide instructions on how to replace the breakthrough filter before the batch was lost.
Some anomalies are much more difficult to detect and predict. In certain situations, for example, an anomaly might occur even when a process appears to be functioning normally. For these more involved cases, data scientists can apply a machine learning technique known as an anomaly detection model. It can identify rare items, events or observations that deviate from the rest of the time-series dataset and are known to be outside normal operating behavior. They can be used to set up monitors and alerts when special deviations occur.
At one plant, fluid from one process occasionally leaked into a compressor and damaged it. This led to a full-plant shutdown and significant loss of production. Engineers determined vibrations were causing the leak, but they had no way to monitor for this problem to occur. Furthermore, operational experts could not intervene in time to prevent damage and avoid an expensive shutdown. Using advanced industrial analytics, engineers collaborated with data scientists to create soft sensors that could detect the anomaly. When it did detect it, they received an alert with instructions and enough time to fix the leak before full shutdown.
Analytics help engineers perform maintenance
As predictive and prescriptive maintenance have become hot topics, engineers have learned that advanced industrial analytics helps them perform maintenance with the right steps at the right time. By using all available data resources and the insight of local experts and central data teams, advanced industrial analytics provides a way to evaluate historical events within their operational context and make predictions about future process behavior. It also creates prescriptive measures to provide instructions for maintenance teams.
By using advanced industrial analytics, engineers can schedule maintenance at the right time along with the right instructions for driving operational efficiency to improving the bottom line.
ABOUT THE AUTHOR
Fréderick Motte is a chemical engineer and the head of customer success at TrendMiner. Motte works to drive value through advanced industrial analytics while bringing exceptional customer experience to process manufacturers.