This guide breaks down the path to maintenance maturity, helping leaders understand where they stand today and what steps they can take to build a smarter and more efficient operation.
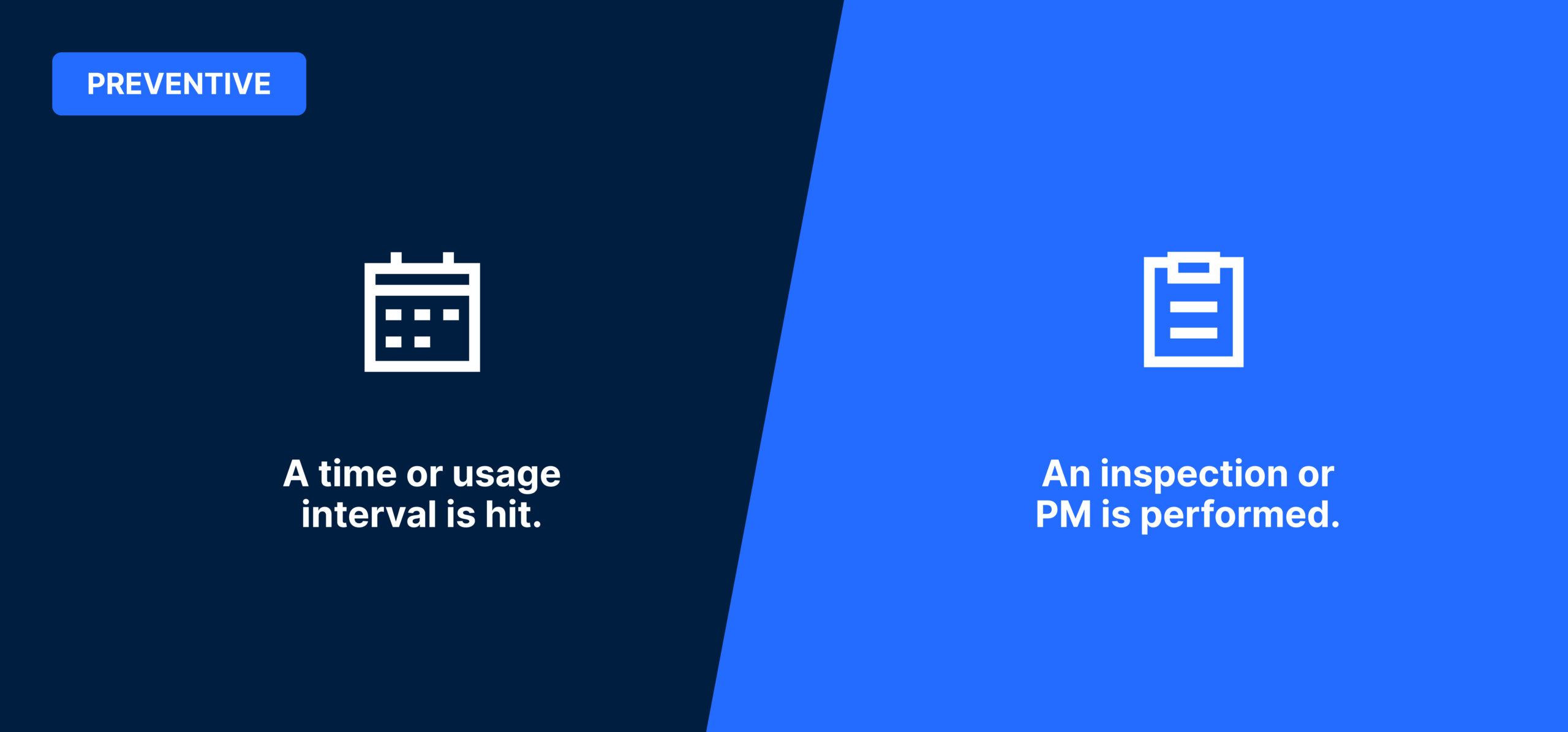
Learning Objectives
- Understand the stages of maintenance maturity: Learn how organizations progress from reactive to prescriptive maintenance and why each stage impacts efficiency and costs.
- Identify strategies for furthering maintenance maturity: Discover practical steps teams can take to capture data better, improve workflows and implement smarter maintenance approaches.
- Recognize the business impact of maintenance maturity: Gain insight into how improving maintenance practices can reduce downtime, cut costs and increase overall operational resilience.
Maintenance insights
- Most maintenance teams want to be more data-driven, but getting there can feel overwhelming.
- In this article, we’ll look at how plant managers can evaluate a maintenance maturity and what the next moves should be in building a smarter, more efficient factory.
The ability to make data-driven decisions is what separates leaders from laggards for maintenance teams. At the highest performing companies, this capability is woven into their culture of excellence. By putting the right information in the hands of their people, these organizations create a foundation for continuous improvement.
So what can companies do to become more data-driven? From artificial intelligence (AI) to automation, there’s plenty of hyped technology out there and a lot of pressure to adopt it.
But the key for maintenance and reliability leaders is not staying on top of trending technology — it’s getting to a place where plant managers and maintenance leaders can take advantage of it.
Every maintenance team is at a different stage of maturity, so there’s no one-size-fits-all approach to modernizing operations. Leaping ahead to industry 4.0 technologies like machine learning and predictive analytics without a strong data foundation in place is unlikely to get results.
The good news is that even if industrial plants feel behind the curve, there are practical steps to take to prepare the maintenance department for innovative tech.
What is maintenance maturity?
A maintenance maturity model conceived in 1999 by manufacturing consultant Winston Ledet still stands as the blueprint of progress in industry maintenance today.
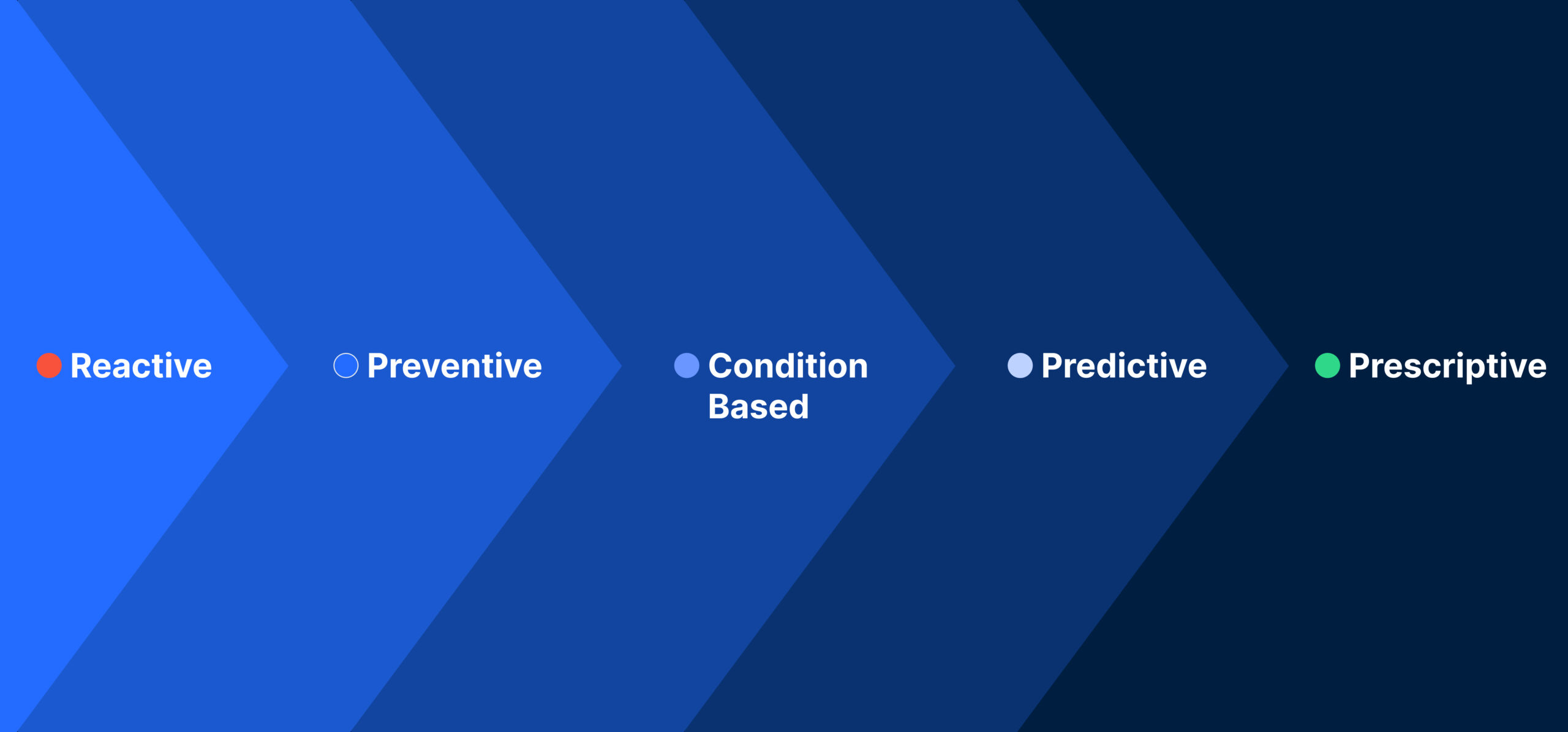
It represents an evolution of handling and maintaining assets to bolster productivity and enhance operational efficiency. There are five levels for companies to progress through: reactive, preventive, condition-based, predictive and prescriptive.
It’s not an entirely linear journey because most companies deploy a mix of these strategies. More critical equipment requires more advanced strategies and these tend to carry a higher upfront cost.
But in essence, the smarter and faster a maintenance team becomes at using data to make decisions, the more “mature” we can say it is. Industry 4.0 technologies like internet of things (IoT) sensors, automation and AI, are enabling higher levels of maturity for today’s most innovative companies.
The business impact of maintenance maturity
The impact of maintenance maturity on a company’s bottom line is significant.
A study by the U.S. Department of Energy suggests companies can reduce their maintenance costs by up to 18% by transitioning from reactive to preventive maintenance (PM). Predictive maintenance (PdM) can yield further savings of up to 12% over basic preventive strategies.
Mostly, it comes down to how soon equipment faults can be detected and how quickly those faults can be corrected before they lead to more costly repairs and breakdowns.
Reactive maintenance
Reactive maintenance, often called “run-to-failure” maintenance, involves addressing equipment issues only after they arise. This can be practical for noncritical or low-cost assets that aren’t considered worth the additional overheads of PM.
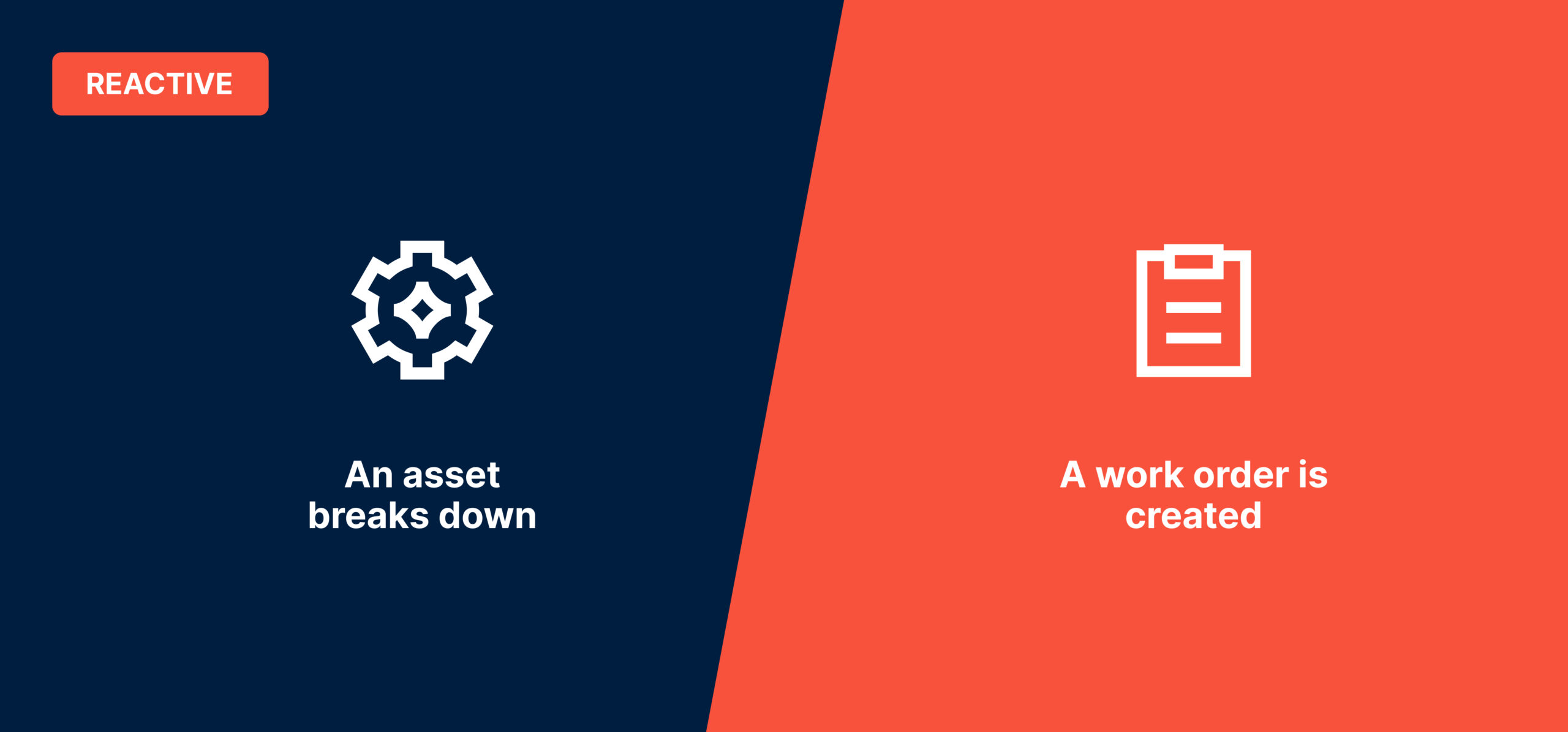
But highly reactive strategies often lead to increased downtime and safety risks due to unplanned breakdowns. The cost to many companies is in lost production revenue. In certain industries, like pharmaceuticals or oil and gas, sudden failures can lead to regulatory shutdowns and even risks to public safety or the environment.
Reactive maintenance is also unpredictable, which makes it hard to keep critical spares in stock and allocate labor resources in advance. This pretty much always results in higher costs through emergency shipping and overtime.
According to industry experts, less than 20% of industrial maintenance efforts should be dedicated to reactive strategies.
Recommendations for teams that are predominantly reactive:
- Focus on prioritization. Deciding which work requests to tackle first is the most basic step in progressing from reactive maintenance to more proactive strategies. Understanding which assets are most critical to production and categorizing them accordingly in the computerized maintenance management system (CMMS) or enterprise asset management (EAM) will keep teams focused on the most important work. Using digital procedures and notifications will also empower technicians to react quickly and bring down mean time-to-repair (MTTR).
- Start incorporating original equipment manufacturer (OEM)-approved maintenance plans. Once the maintenance team has highlighted the most critical equipment, it can start incorporating PM plans from OEMs. This will extend the machines’ operational life and unearth issues that can be corrected before they take down the production line. Modern CMMS platforms allow experts to download OEM-approved PM plans for equipment instantly, which can really kickstart a preventive strategy.
- Capture higher quality work and asset data. Most maintenance teams running their equipment to failure have very little data they can use to plan. They often have a gut feeling about which equipment breaks down the most, but they can rarely say with confidence why these breakdowns happen or how much they cost to fix. Making it easy for technicians to record what went wrong and what they did about it is the first step to building a data foundation that enables all the remaining stages of the maturity model.
Preventive maintenance
PM involves scheduling regular inspections, servicing and minor repairs to prevent equipment breakdowns. In many industries, PM also helps companies stay compliant with regulations by ensuring that equipment is clean and safe to use.
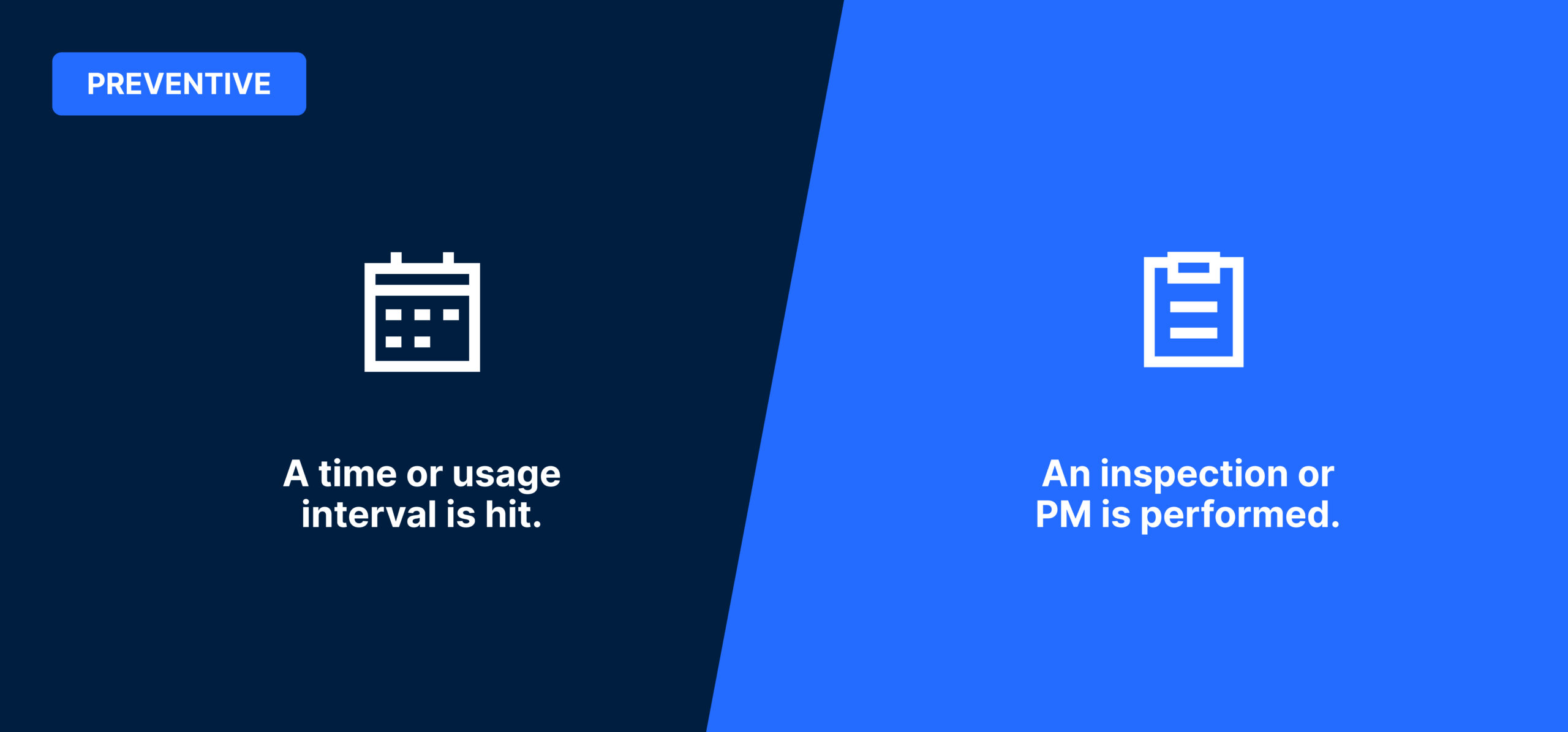
While PM appears more costly than reactive — because labor hours are spent whether the machine is broken or not — it pays back to the business by preventing more costly downtime incidents. Studies have shown that every dollar spent on PM can prevent $5 in future expenses to the business. Chemicals and plastics manufacturers rely on PM to prevent defects by ensuring machines are lubricated and aligned.
Basic PM involves performing maintenance on a time schedule, or after a certain number of miles or run hours. Although this is more cost-effective than reactive maintenance, it’s not well optimized. Equipment still fails unexpectedly and teams often overspend on unnecessary maintenance out of caution.
Recommendations for teams doing basic PM:
- Use data to adjust PM schedules. OEM manuals are a good place to start when assigning PMs to machines. But there’s only so much room to optimize a program using the data in maintenance software. Asset health reports in a CMMS can highlight which components need more diligent inspection — while managers might also discover that they are doing more PM than necessary on certain equipment. Tracking MTTR and mean time between failure will also inform stronger PM plans.
- Standardize workflows. Implement digital standard operating procedures (SOPs) to make sure everyone is following the right steps and tracking them all in the same way — even across facilities. This helps leaders evaluate teams and processes, implement improvements and collect rich maintenance records that can inform predictive models in the future. Modern maintenance software can help generate SOPs quickly using AI.
- Start condition monitoring for the most critical assets. The best way to optimize a preventive strategy is to listen to the machines. It’s easier than you might think to attach temperature or pressure sensors and start automating readings in a CMMS. This will equip manufacturing teams to get ahead of breakdowns and perform more cost-effective maintenance, by detecting abnormal conditions and performing maintenance only when needed.
Condition-based maintenance
Condition-based maintenance (CbM) is a strategy that monitors the actual condition of an asset to decide when maintenance is needed.
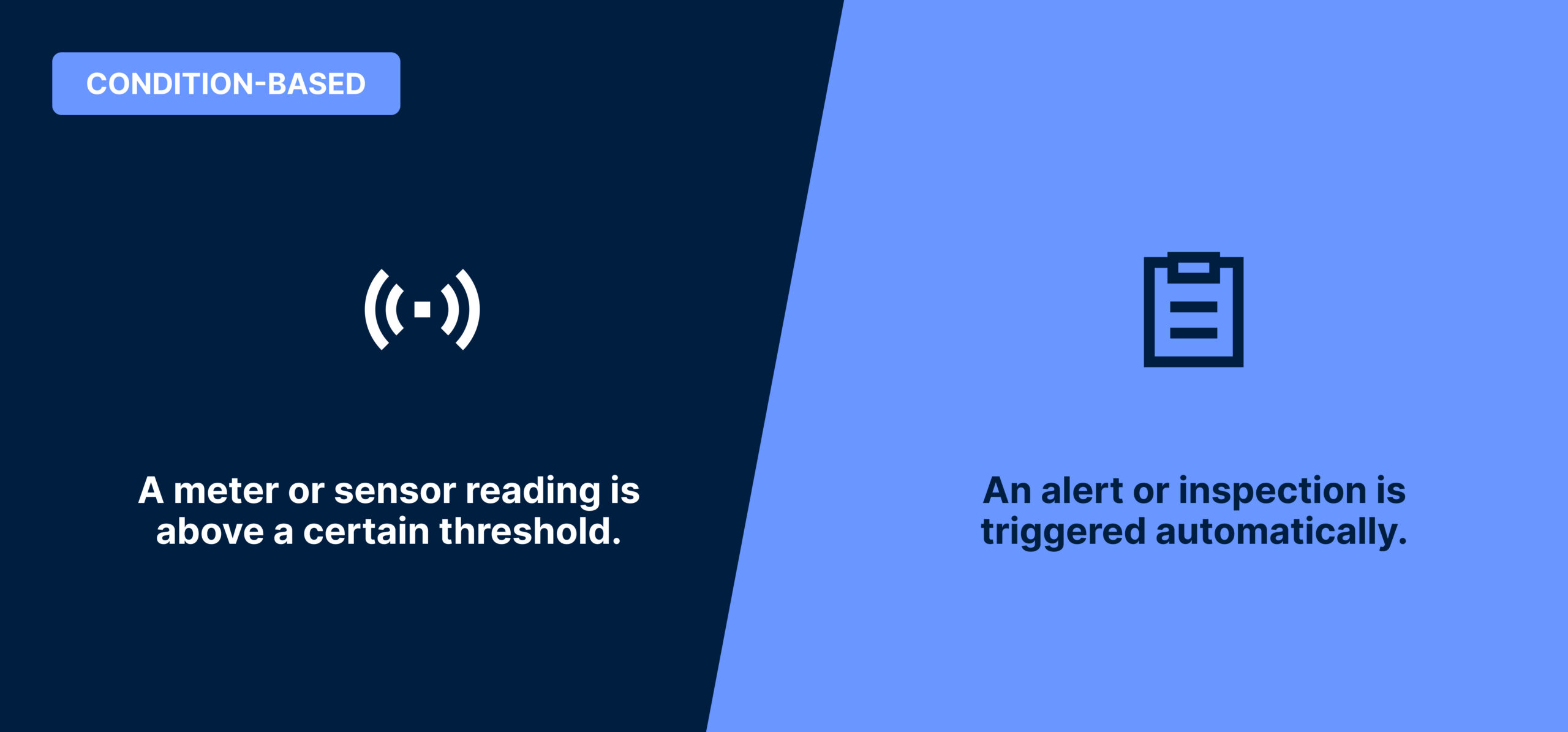
This requires teams to capture real-time data on temperature, pressure, energy usage and more and check for abnormal values. Readings can be taken manually in some cases, but usually condition monitoring programs use IoT sensors to send condition data to a CMMS or EAM automatically, where it is used to trigger work orders and notifications.
Mining companies use vibration, temperature and oil analysis sensors to prevent catastrophic failures in haul trucks and crushers. Pharmaceutical plants monitor air filters and pressure differentials in cleanrooms to detect inefficiencies and contamination.
CbM is a step forward in maintenance maturity because it enriches a company’s maintenance records with real-time equipment data. Upfront costs are often the barrier to adopting it. But in the long term, CbM can significantly reduce maintenance costs by eliminating unnecessary PM and catching faults before they take down production lines.
Recommendations for teams with CbM programs:
- Use automations to streamline CbM workflows. Condition monitoring is a powerful tool, but the data isn’t valuable unless it can be quickly acted upon. CMMS automations trigger work orders and notifications automatically when predetermined condition thresholds are hit. For more simple monitoring like temperature and pressure, this relieves the team from having to analyze any data in-house — empowering them to simply act when needed.
- Connect operational technology to get more context. Wireless sensors are just one of the many data sources that can inform condition-based workflows. Connecting programmable logic controller PLC and supervisory control and data acquisition systems to the asset management software can provide automated insights on runtime, energy consumption and failure codes, which inform t maintenance team when machines are malfunctioning or have dipped in efficiency.
- Adopt vibration monitoring to start predicting failures earlier. More advanced condition monitoring tracks small changes in vibration to detect faults long before they take the equipment down. Vibration monitoring identifies early warning signs like worn bearings, misalignment and imbalance. It’s one of the best ways for a company to take its CbM program to the next level and start PdM.
Predictive maintenance
PdM represents a significant leap in maintenance maturity. While CbM is about reacting to clear signals that maintenance is needed, PdM uses advanced analytics and machine learning to forecast these needs ahead of time.
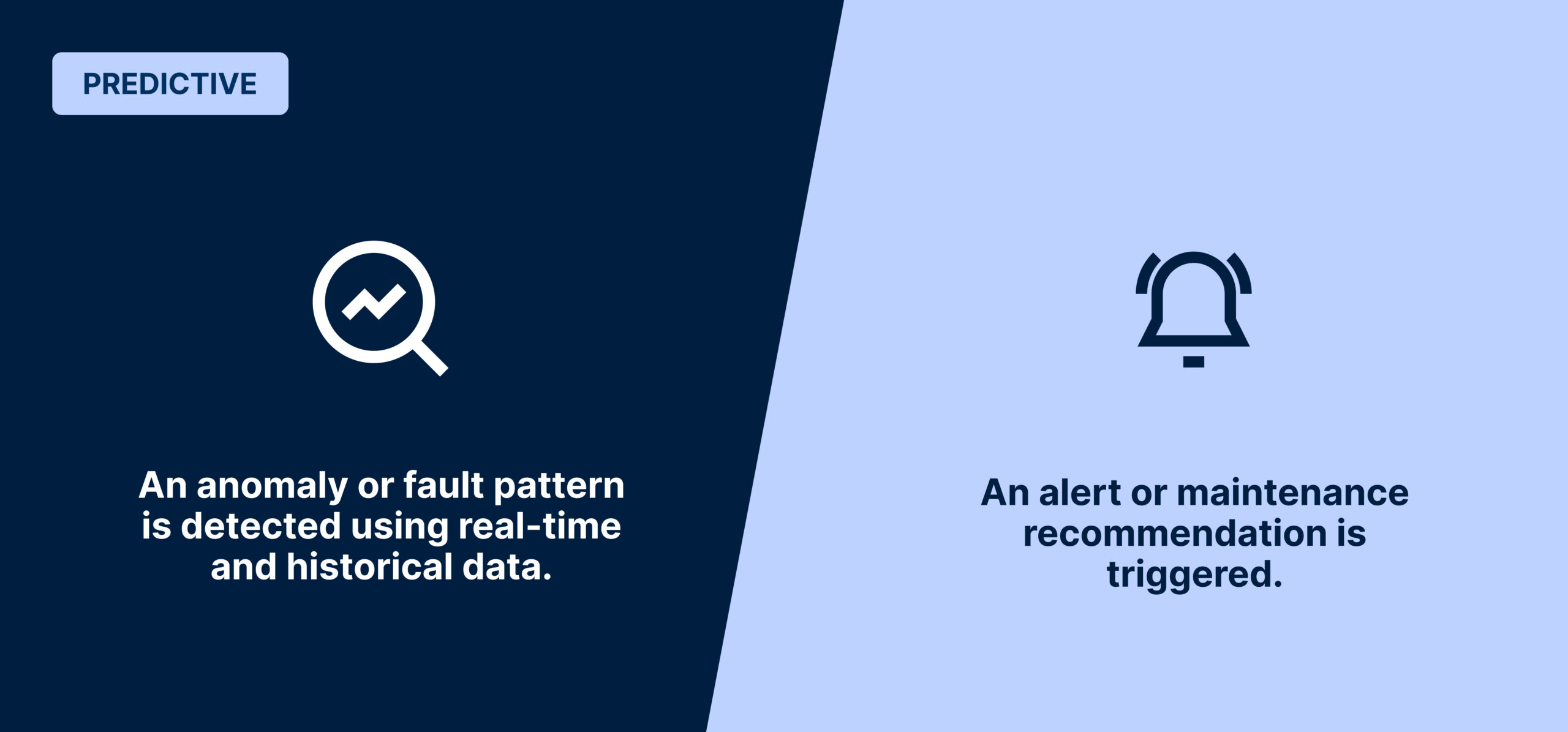
This level of insight allows organizations to optimize resources and reduce breakdowns by up to 75%, according to Deloitte. A good example is monitoring the vibration of an electric motor driving a conveyor belt in a food processing plant. Unbalanced rotors and worn bearings can be detected very early, avoiding most costly maintenance in the future.
Because PdM leverages historical as well as real-time data, it relies on high-quality maintenance records to be effective. It’s critical for maintenance teams to start building this data foundation today, even if they’re not yet at this stage of maturity.
Recommendations for teams doing PdM:
- Evaluate data quality. Some companies don’t get the value they expected from PdM — and it often means they need to assess the quality and reliability of the data feeding those models. The richer and more standardized records are, the better these models will work. Consider diversifying condition monitoring to include vibration, temperature, pressure and energy usage. This builds a more comprehensive picture of the equipment’s health. Focusing on most critical assets first makes this easier to achieve.
- Make insights more actionable using AI and automation. PdM can also fall short when teams fail to bridge the gap between insight and action. Technicians need to know how to act on predictive insights for them to be useful. Triggering work orders and notifications automatically turns vibration analysis into clear action steps. Advanced analytics and AI tools can help maintenance teams identify patterns, catch anomalies early and make more informed scheduling decisions.
Prescriptive maintenance
Prescriptive maintenance is the highest level of maintenance maturity. In this strategy, machine learning not only delivers predictive insights, but informs the planning, scheduling and execution of maintenance work as well.
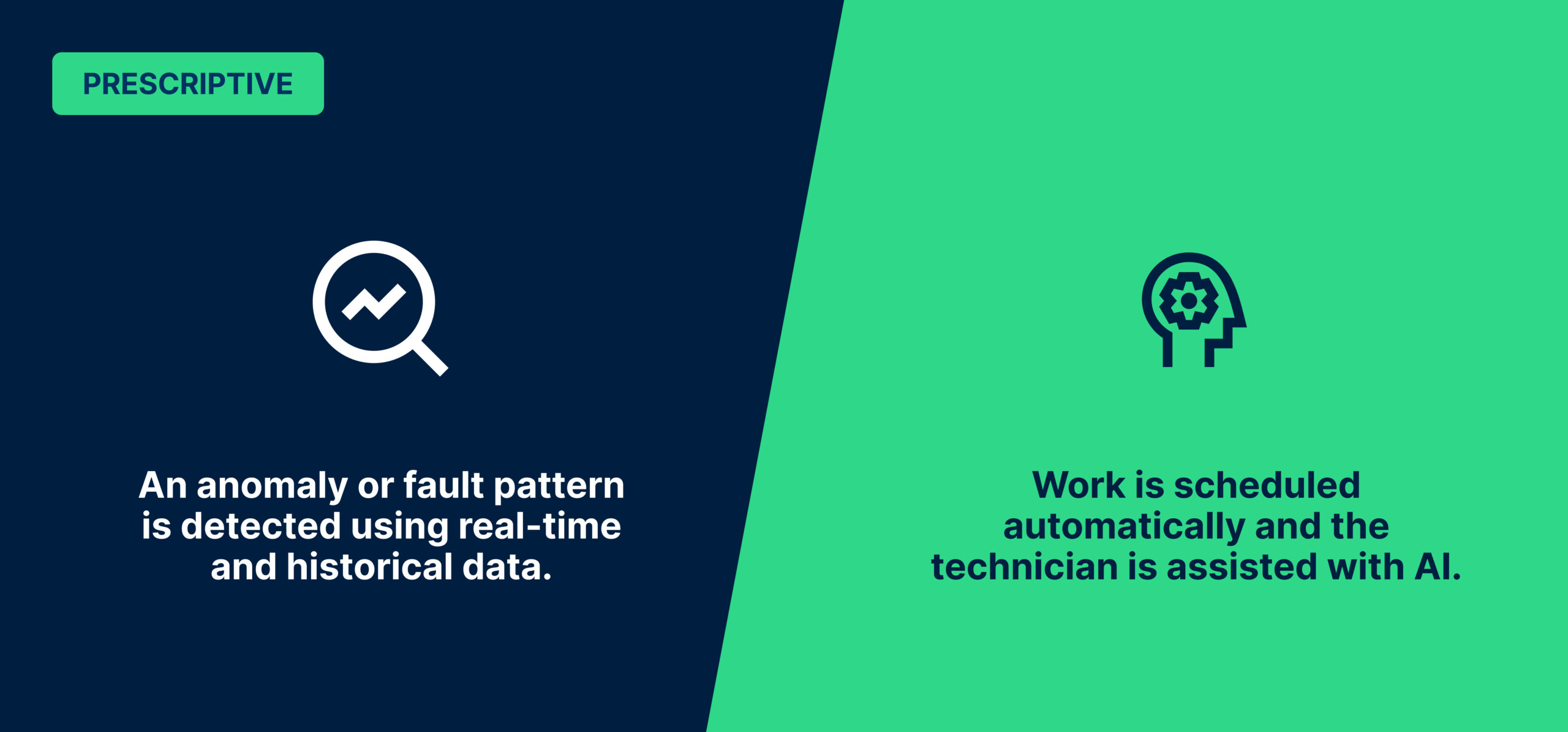
While PdM unearths equipment issues early and raises the alarm, prescriptive maintenance suggests repair steps and parts, even going as far as assigning a technician automatically based on their experience performing similar jobs.
AI-powered insights assist the technician as they complete the work and then the details of that job are fed back into the predictive models to inform stronger recommendations the next time around.
While this strategy might feel unrealistic to many companies today, the technology is available and rapidly improving. The key for maintenance leaders is to make it as easy as possible for their teams to collect high-quality data, so that AI and automation can be adopted successfully.
Building enduring maintenance plans
With rapid advances in technology and increasing pressure to digitize operations, maintenance leaders often feel overwhelmed by the path forward. But the journey to maintenance maturity isn’t about chasing the latest innovations — it’s about building a strong foundation of standardized processes and high-quality data.
Success comes from taking measured steps: documenting processes consistently, collecting reliable data and gradually introducing more advanced monitoring and analytics capabilities. By focusing first on the fundamentals, maintenance teams can create the operational excellence that makes advanced technology truly valuable.
The most successful organizations recognize that maintenance maturity is an evolution, not a quick U-turn. They empower their teams with the right tools and training, measure results carefully and scale what works. In doing so, they build not just better maintenance programs, but more resilient and competitive organizations.