Process manufacturers are combining retrospective analysis with predictive tools using advanced analytics platforms, empowering them to build models, project failures, determine optimal maintenance schedules and increase uptime
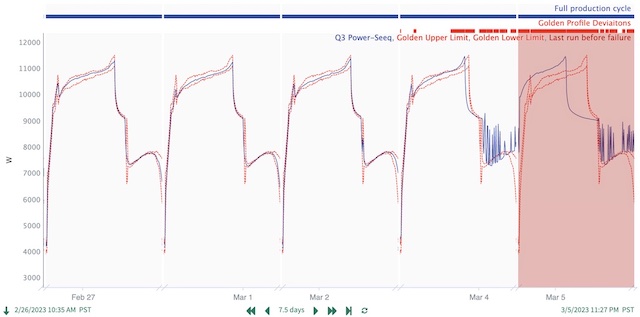
Learning Objectives
- Understand different modifiers that qualify “analytics,” especially retrospective versus forward-facing descriptors.
- Recognize the importance of full data context for solving complex process problems.
- Apply advanced analytics to transition maintenance programs from preventive to predictive.
Advanced analytics insights
- Advanced analytics, employing sophisticated methods like machine learning and artificial intelligence, empower organizations to convert historical, real-time and predictive data into actionable insights, thereby significantly enhancing decision-making processes.
- This proactive approach allows manufacturers to anticipate potential issues and implement timely measures, ultimately improving operational efficiency and minimizing unexpected downtime.
Across the process industries, advanced analytics can guide organizations through fruitful, problem-solving journeys by examining historical, current and predicted time series data, which unveils insights that drive improved decision-making.
In the past, process engineering teams relied solely on stored data to inform process changes focused on preventive maintenance, mitigation strategies and optimization initiatives for improving operational efficiency. These efforts were conducted with an emphasis on monitoring, centering on the most recent operations data available to identify problems.
As the explorations progressed organizations dove into data stored in process historians and other databases to fine-tune insights, using diagnostic analytics to investigate recent problems, such as deviations during a prior batch.
To increase efficiency, process manufacturers must shift from reactive approaches — responding to problems as they arise — to proactive approaches, which leverage historical data and context to drive more accurate process improvement decisions. Modern advanced analytics platforms are empowering these manufacturers to implement proactive measures that anticipate and address potential issues before they swell into larger process problems, providing greater operational efficiency, higher performance and increased uptime.
Understanding advanced analytics
“Analytics” is a general and broad word, typically linked to software products, platforms and the cloud. Therefore, it must be qualified with modifiers.
For example, advanced analytics describes the use of statistics, machine learning and artificial intelligence in data analysis to assess and fine-tune insights. Other modifiers can be used to differentiate the analytics type based on utility and complexity.
For instance, diagnostic analytics describes the use of information from past events to respond to a given situation, investigating a raw set of historical data and applying statistical analysis to identify patterns and produce insights. With this approach, teams are often faced with an inescapable lag between the event or issue under analysis and the action taken to improve future performance, eliminating the ability to prevent damage outright.
Descriptive analytics also look at the past, with the goal of summarizing events via reports that teams can easily interpret and learn from. In this practice, the same lag occurs and it can be difficult to develop clear-cut answers on how and when to make decisions that will prevent future performance issues.
While both retrospective methods provide value, they fail to solve the whole puzzle. Predictive analytics provides the missing piece by using historical data to develop and train models that project future data, enabling teams to foresee what will happen, when it will happen and the actions they should take to drive desired outcomes.
Leveraging advanced analytics in maintenance
Before looking ahead to future system behavior, an engineer must understand past and present operating conditions. This is best accomplished when equipped with a live connection to all relevant processes and contextual databases.
Industrial organizations can leverage advanced analytics platforms to connect disparate data sources to a central cloud-based source, alleviating the challenges of live data connectivity, such as retaining multiple logins or constantly bouncing back and forth between interfaces. These platforms provide subject matter experts with simplified data-cleansing tools and contextualization, enabling them to rapidly derive meaningful and reliable insights from all available data.
Once live connections are made, teams can start their journey to valuable predictions, including equipment-based predictions — or predictive maintenance — where the objective is to predict when equipment will require maintenance or fail. While techniques vary, the goals of predictive maintenance — increasing quality, reliability and uptime — are fixed.
Advanced algorithm functionality drives an advanced analytics application’s predictive capabilities in which the application matches an organization’s specific workflows and procedures to a respective law, theorem or process design principle to create key performance indicators. This enables accurate data extrapolation when expanding future timeframes for insight and analysis.
The following sections detail situations where advanced analytics helped process manufacturers enhance operational efficiency and pivot from preventive to predictive maintenance approaches.
Using advanced analytics for valve erosion prediction
Valves are found in almost every industrial facility, playing critical roles in process control. Keeping these assets in top condition is essential for maintaining efficient operations, but condition monitoring can be tricky based solely on observation. Advanced analytics solutions often provide low-effort, high-return opportunities to monitor valve conditions and reduce unexpected failures, simultaneously protecting adjacent process equipment and devices.
An oil and gas producer operating more than 50 well pads — each with a gathering system containing a critical flow control valve — was experiencing frequent valve failures. Each failure occurrence rendered the pad inoperable for days until repairs could be made. These failures were typically caused by sand erosion, and the producer had no methodology in place to determine early warning signs other than exhaustive manual inspections, which required complete shutdown and became increasingly cost-prohibitive as the asset base grew.
By deploying an advanced analytics platform to monitor process conditions, the producer was empowered to predict erosion progression and approximate the time to valve failure. To establish this analysis, subject matter experts leveraged both real-time and historical process data to calculate a metric indicating progressive erosion in the valve seat, thereby establishing an indicator to predict future failures. The team leveraged first principles to produce this metric, which was used as the basis for a predictive model to approximate time to failure.
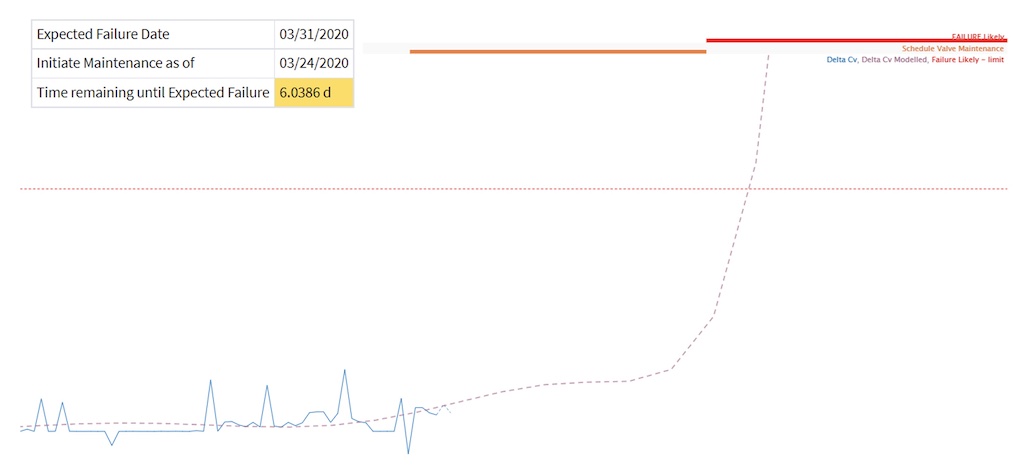
This analysis was scaled to all well pads by leveraging historian hierarchies imported into the analytics platform through its native connectors. The ability to forecast failures enabled the maintenance team to prioritize service, significantly reducing downtime and operating expenditures.
Compressor health monitoring and maintenance
Detecting and predicting compressor performance issues, which can cause sudden and catastrophic shutdown or environmental safety concerns, is another common area for applying predictive maintenance strategies. Advanced analytics can be used to monitor compressor health variables to detect poor performance and mechanical degradation without tedious time spent examining data in spreadsheets.
In one large manufacturing facility, data scientists leveraged machine learning algorithms to drill down to the root cause of compressor failure. Next, they superimposed the algorithm on live data to identify signs of degrading performance. Subsequently, they used their findings to create maintenance notifications ahead of failure, which provided insights on a visual interface for operators and process engineers.
These types of tools empower engineers to identify leading and lagging indicators of degrading compressor health and to continuously monitor variables, helping teams proactively identify risks and prioritize maintenance activities.
Reactor failure mitigation
Collaboration between teams — such as process, maintenance and reliability — can be strengthened by leveraging built-in tools within advanced analytics platforms for sharing analyses and insights in easily digestible dashboards and reports.
One petrochemical and refining company was experiencing significant reactor shutdowns caused by a failing critical feed gas compressor on a polyethylene line, and these impactful failures precluded any way to immediately restart the process. For this facility, an unplanned reactor shutdown causes a minimum of four hours of downtime, costing the plant upward of $200,000 with every incident. Previously, these compressors had been maintained on a preventive maintenance schedule, but this did not prevent unplanned shutdowns entirely.
Following one compressor trip, machinery and controls engineers worked together to identify the safety interlock that prompted the shutdown, bringing electrical engineers in to assist with the investigation. However, tracing electrical diagrams around the pump motor was time-consuming — and it failed to yield a root cause.
A process engineer at the refinery took an alternative approach, using an advanced analytics platform to rapidly locate the five most recent shutdowns and subsequent restarts — planned and unplanned — from decades of historical process data. With time-dissection tools, they focused on shutdown and startup time periods and overlaid all events, presenting abnormalities in the discharge pressure profile of the two most recent startups.
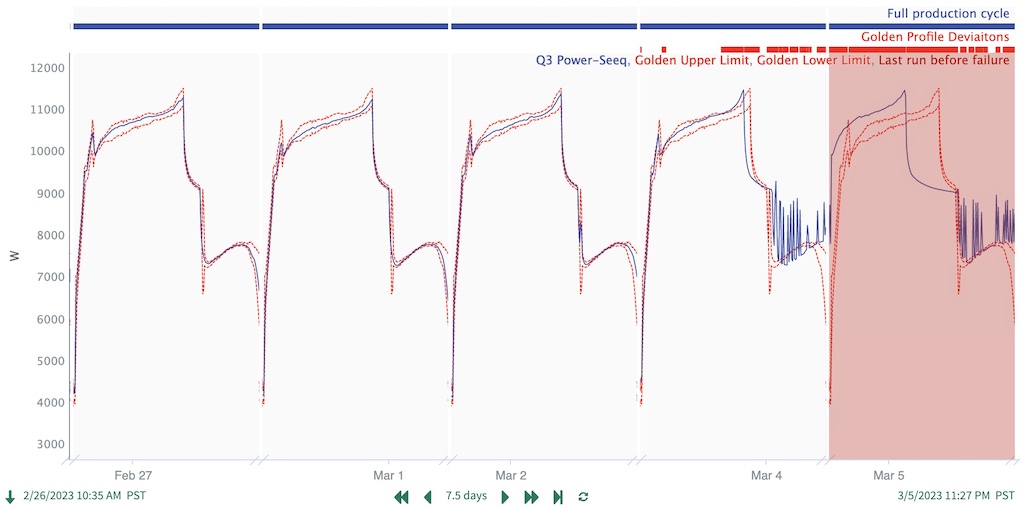
Upon further investigation, the engineer also identified early warning signs on the motor amperage signal. Without a method to view the startups back-to-back, the motor degradation had gone unnoticed by operations.
As a result of this root cause analysis, the process engineer implemented a monitoring solution to identify and flag future motor degradation to prevent similar unplanned shutdowns. When an out-of-tolerance value appears, the compressor motor is now immediately added to the maintenance work list for the next planned shutdown, a proactive maintenance approach that is expected to eliminate unplanned shutdowns due to this failure mode.
Making advanced analytics work for you
Accurate process manufacturing predictions rely on in-depth knowledge of past equipment behavior and outcomes. By using advanced analytics platforms to combine retrospective with predictive analytics, process experts and data analysts can easily build robust models, capable of predicting plant maintenance needs and risk-mitigating procedures.
With the right tools in their digitalization toolbox, process manufacturers can build better models to provide vast plant insights and project issues before failure so personnel can optimize maintenance schedules and prevent costly downtime.