Advanced analytics platforms empower maintenance transformation through predictive modeling, helping manufacturers shift from reactive to proactive approach.
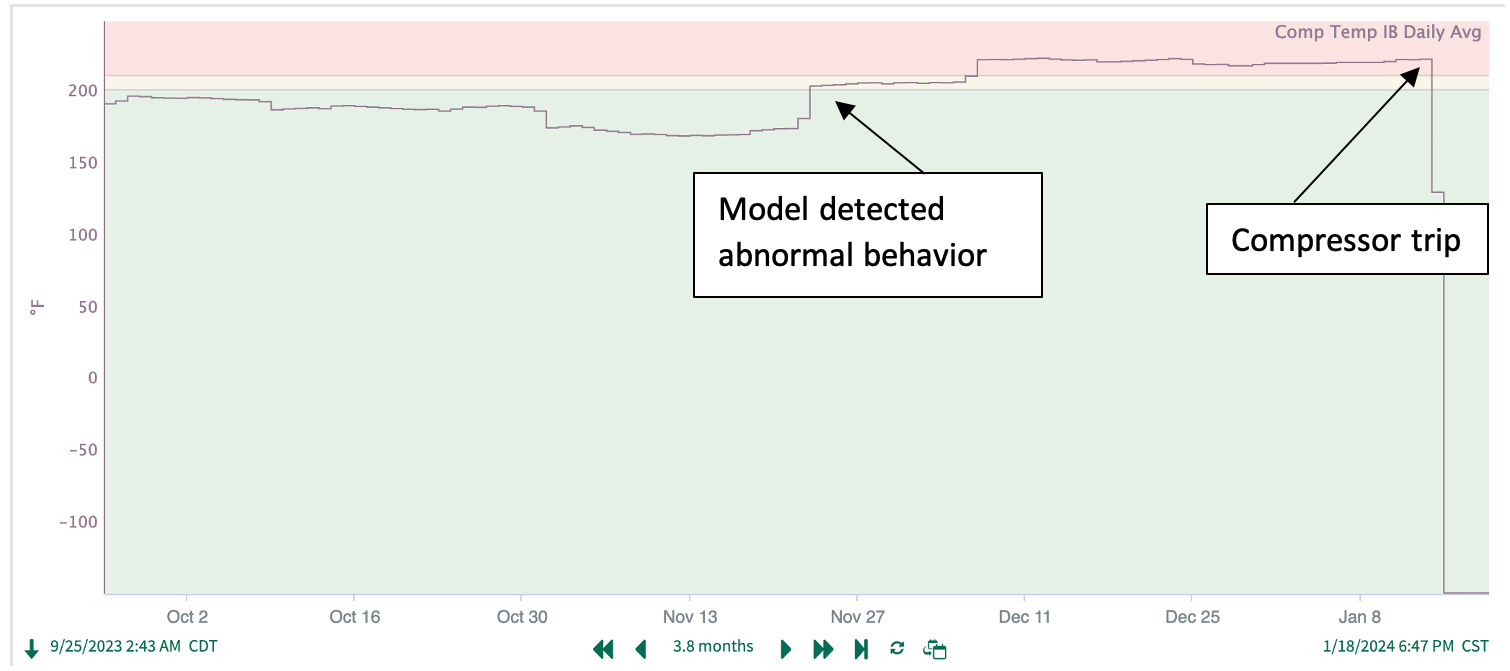
Learning Objectives
- Understand the difference between reactive (run-to-failure) and proactive (preventive and predictive) maintenance.
- Learn strategies to efficiently create and verify predictive models.
- Apply advanced analytics platforms for data aggregation, insight creation and modeling.
Predictive maintenance insights
- Sound maintenance procedures are critical for manufacturers to ensure safe, efficient operations and reduce costly unplanned downtime, which can cost the entire industry up to $50 billion annually.
- By leveraging predictive maintenance strategies and advanced analytics, manufacturers can proactively address equipment issues, improve asset reliability and increase profitability while meeting safety and environmental standards.
In every plant throughout every industry, sound maintenance procedures are vital to ensure safe and efficient manufacturing operations. An average manufacturer faces more than 800 hours of equipment downtime per year and unplanned outages cost the industry as much as $50 billion per year globally.
Asset-intensive industries in particular — such as oil and gas, chemicals, pharmaceuticals, mining and power — rely on heavy equipment for day-to-day operations and unplanned outages can wreak havoc on the bottom line. By keeping equipment running reliably, manufacturers can minimize surprise downtime and increase production, quality and throughput while adhering to safety and environmental standards.
However, achieving this requires thoughtful maintenance planning and practices.
Preventive maintenance procedures, which require servicing equipment at regular intervals, gained prominence decades ago and opened the door to proactive maintenance strategies. This provided unquestionable upgrades over reactive run-to-failure methods, but the approach introduced new costs in the form of unnecessary maintenance or simply inefficient allocation of technicians’ time-maintaining assets without any need for service.
The rise of continuous and automated monitoring technology in more recent times ushered in a new era of proactive maintenance efficiency, employing predictive maintenance procedures to guide plant upkeep efforts. This is accomplished by using both real-time and past performance data to foresee potential equipment issues before failure and then proactively schedule at-risk components for maintenance.
The run-to-failure mindset is actively being replaced by intentional inspection strategies, critical sparing programs and data-driven approaches to prioritize not only what work gets done in the field, but also how it is executed. By leveraging digital tools and employing these methodologies to shift from reactive to proactive maintenance, leading organizations have reduced unplanned downtime and improved maintenance labor productivity, resulting in profitability growth by up to 10%.
Identifying opportunities for predictive maintenance
Predictive maintenance strategies are effective for preemptively identifying signs of equipment degradation, ineffective energy use and other negative process implications for both rotating and static equipment.
In many manufacturing environments, a single engineer or maintenance manager is responsible for hundreds, if not thousands, of assets. With such a magnitude of equipment, the task to effectively monitor, prioritize, schedule and perform maintenance is daunting without supportive systems in place.
In a recent survey by McKinsey & Co. of senior leaders at asset-intensive manufacturing companies, 99% claim to have embarked on a maintenance transformation journey in the past five years.
However, these initiatives frequently fail to meet expectations due to the large volumes of data spread across disparate sources, a lack of work and workflow management vision and gaps in workforce enablement.
Data plays a critical role in predictive maintenance to monitor the asset health of a unit, plant or even enterprise. This information comes from real-time field sensor data, in combination with retrospective information from process historians, enterprise resource planning, manufacturing execution and other similar database resources.
By aggregating and analyzing this collective information within a powerful advanced analytics platform, eliminating unplanned downtime becomes a more attainable goal for manufacturers. The addition of artificial intelligence (AI) agents to help develop monitoring tools accelerates the technique further by empowering subject matter experts (SMEs) to achieve more in a shorter span of time.
Once predictive monitoring is in place, the next step is to prioritize maintenance efforts to ensure the highest impact equipment — from both safety and profitability standpoints — have proactive maintenance procedures in place. This decreases the probability of an unplanned equipment outage and therefore minimizes the potential consequences, including poor reliability, inability to meet production targets, off spec quality, safety issues and overages in maintenance spending.
Data-driven models for asset reliability
Robust monitoring systems and data access are enabling drivers for data-driven and risk-based maintenance. To detect when an asset is operating outside its typical bounds, normal operating baseline data is needed. For some equipment, such as pumps and compressors, original equipment manufacturers often specify ideal operating limits. In other cases, the normal operating window must be established using historical runtime parameters, with anomalies removed from the baseline. These historical datasets must often contain years’ worth of information to account for seasonal impacts, unit throughput capacity and other changes in operating events.
Developing the right model based on the sensor and process data available requires time from an SME with a strong understanding of the equipment and the overall system. Advanced analytics platforms simplify model creation by empowering teams to filter out downtimes, identify behavior corresponding with previous maintenance events — e.g., just after the last time a heat exchanger was cleaned or following the most recent compressor overhaul — and compare time periods with similar operation. The evolution of AI in process monitoring platforms has accelerated development and scaling of models and algorithms further, increasing insights available while reducing SME time required.
Even with robust models in place, individual equipment strategies are constantly evolving due to other changing conditions within a manufacturing facility. For example, an asset’s criticality calculation may shift with a change in lead time for replacement components, asset health of a spared system or new economic drivers that impact unit profitability. Models that monitor fleet health can provide alerts for engineering, operations and maintenance teams to help them operate equipment properly, prioritize maintenance reasonably and keep production plans on track based on current constraints.
Proactive monitoring for static equipment: heat exchangers
Phillips 66, a large multinational energy company, used Seeq — an industrial analytics, AI and monitoring platform — to develop a monitoring tool for its fleet of brazed aluminum heat exchangers (BAHX). A catastrophic failure occurred in 2016 at another company in the same industry and the U.S. Chemical Safety Board subsequently issued BAHX operating best practices to prevent the future occurrence of similar safety and environmental incidents.
Adhering to these recommended industry standards required monitoring the number of cycles and temperature differential within each heat exchanger, to notify operations staff when limits were exceeded, prompting appropriate action.
By leveraging the thermocouple data from the inlet and outlet of the hot and cold flow streams of each heat exchanger, the approach temperatures and running cycle count were calculated over six years of data for more than 100 heat exchangers. The calculation also accounted for the temperature differential severity because the likelihood of thermal cyclic fatigue at a brazed aluminum joint increases with higher temperature gradient, which can lead to failure.
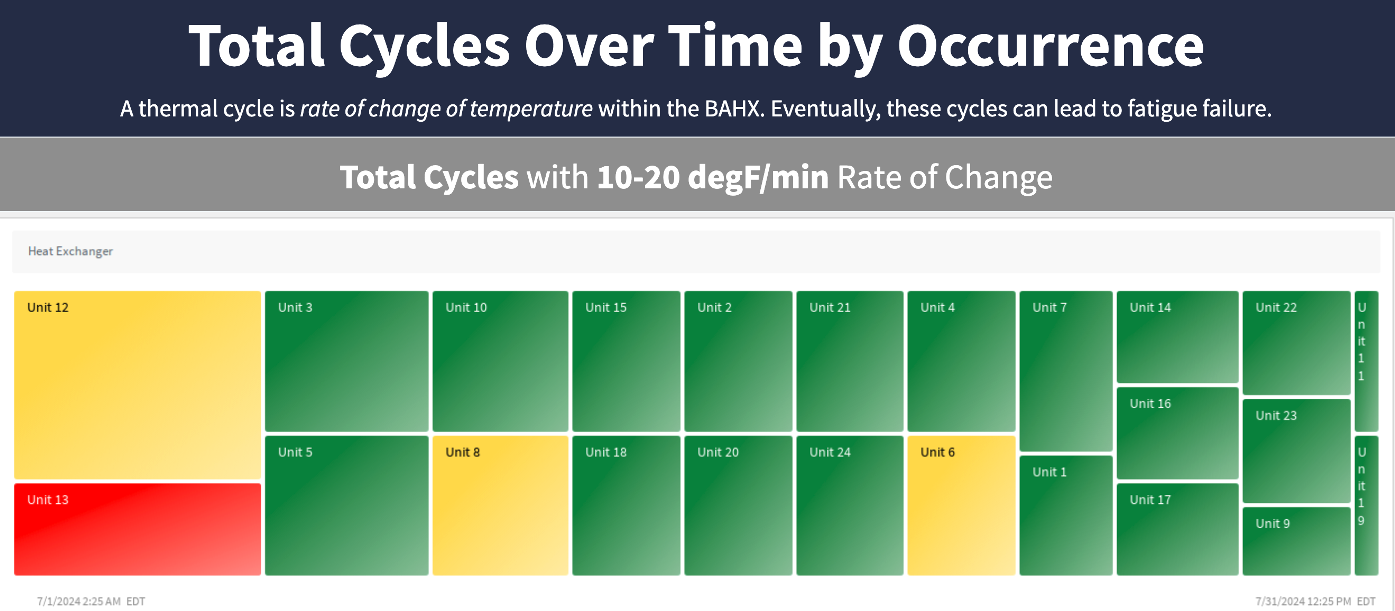
After creating the initial analysis, the team was able to scale the calculations across a custom asset hierarchy to proactively monitor more than 100 BAHX using a dashboard (see Figure 1). Every day, the reliability team receives an asset health report and they can take preventive measures operationally or mechanically to preemptively mitigate the risk of catastrophic failure.
Proactive monitoring for rotating equipment: compressors
Saudi Arabian Oil Co. (Saudi Aramco), one of the world’s largest integrated energy and chemicals companies, used the same advanced analytics platform to predict centrifugal gas compressor failures. Historically, the company monitored temperature and vibration excursions solely in its alarm management system.
However, the team needed a predictive tool to track gradual deviations in compressor sensor data to avoid system trips, rather than the reactive approach of relying on its alarm system.
The predictive models that were developed monitored temperature and vibration to identify abnormal behavior, deviations and aggressive oscillations. First, the signals were cleansed for high-frequency noise, outliers and periods of bad data. Next, baseline behavior was determined by finding a dynamic threshold based on yearly averages with a margin of error. Then, daily average values were compared to the rolling yearly average to identify anomalies and the system generated alarms when abnormal conditions were detected.
This strategy was deployed on a fleet of compressors with more than 600 sensors to give the user enough time to investigate temperature and pressure excursions before a compressor trip. Then, the appropriate actions could be taken by operations and maintenance teams to avoid the trip altogether. The model was validated using historical trip event data to determine whether the exceedances would have been flagged with enough time before the trip and in several cases, anomalies would have been identified a full month before (see Figure 2).
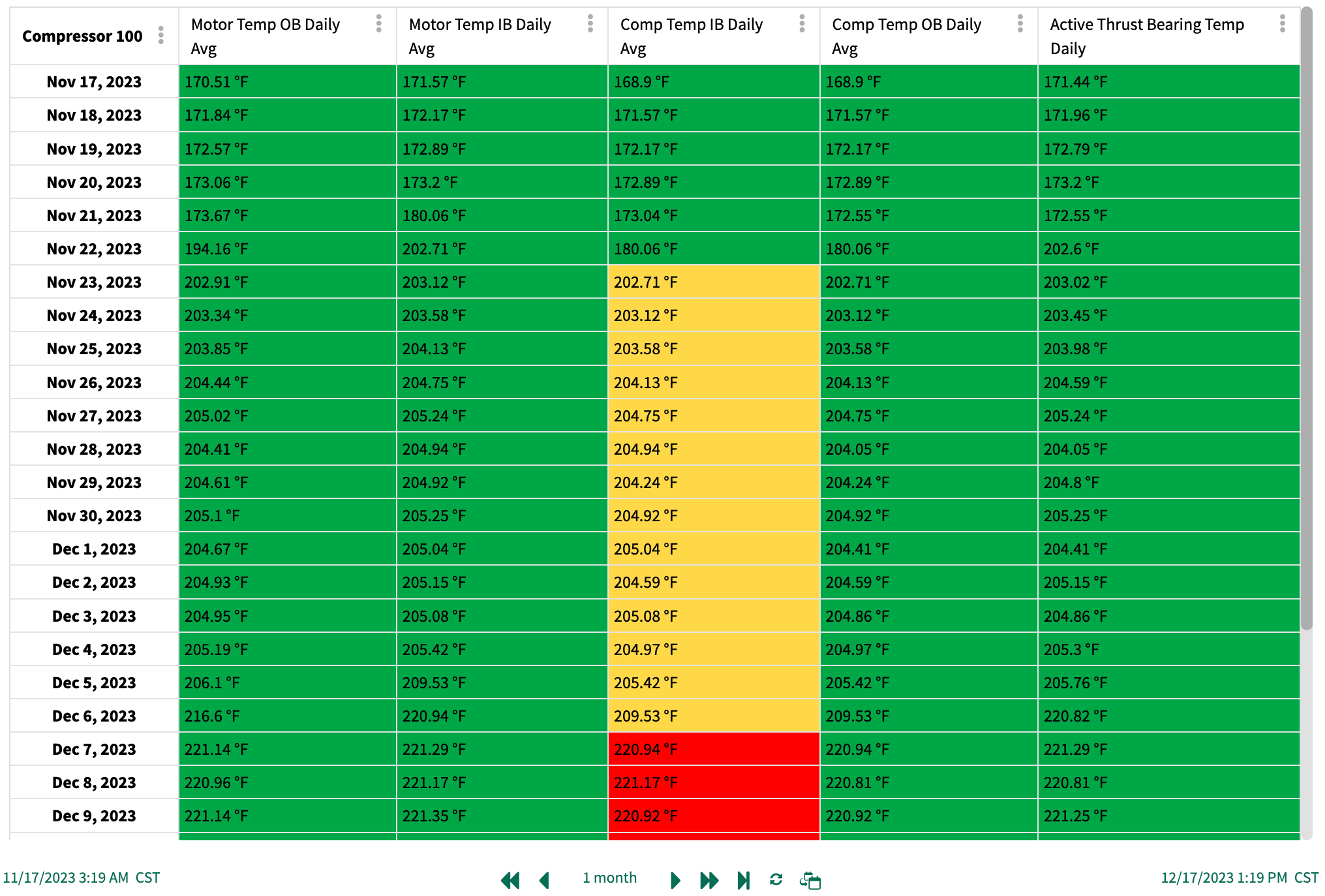
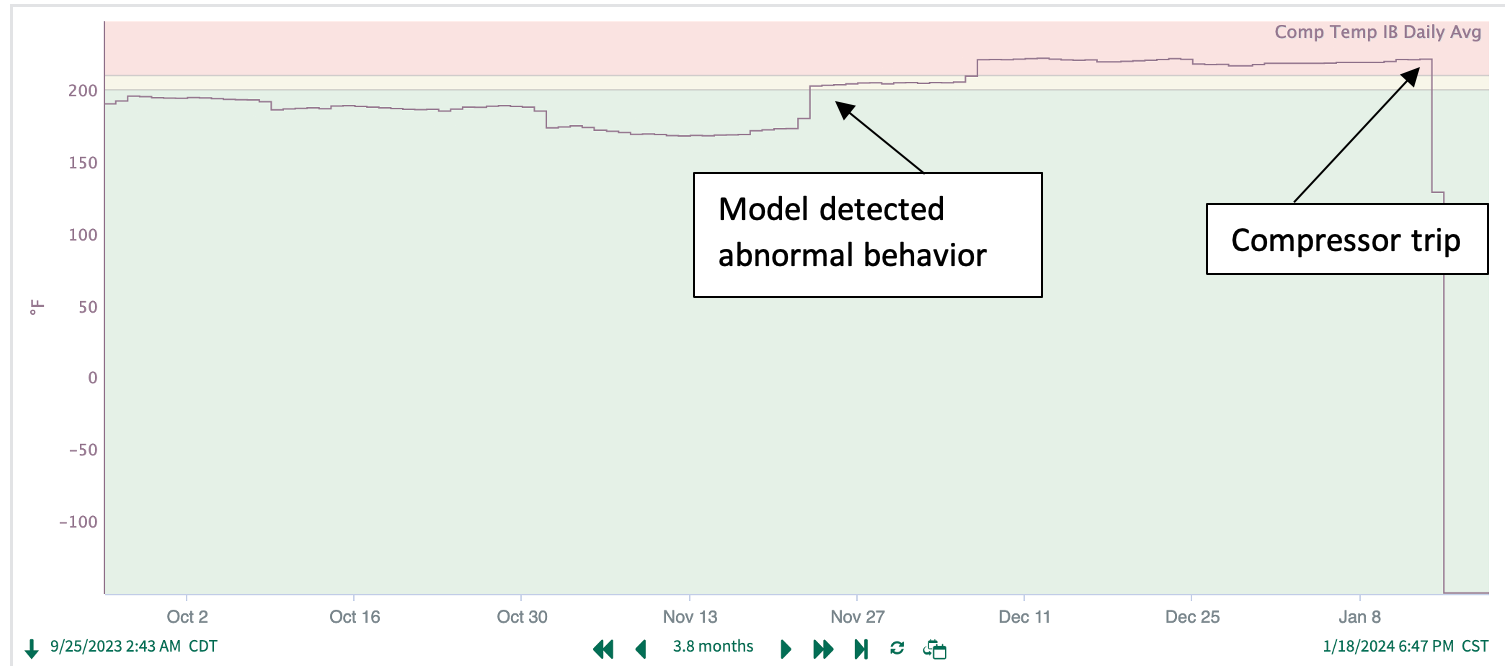
By implementing this tool, the company has saved hundreds of thousands of dollars on reactive maintenance and downtime losses, decreased flaring events and improved the reliability of its compressor fleet. The team monitors all 600 sensors to identify compressor anomalies and predict potential failures.
Transitioning from reactive to predictive maintenance
Many large and small manufacturers in numerous industries have tried and failed to implement predictive maintenance strategies with existing toolsets. The task is incredibly daunting with the complexity and volume of assets that must be monitored.
However, advanced analytics platforms significantly simplify data aggregation from multiple sources and insight creation, providing the building blocks for predictive modeling. With a clear company vision, accessible data, an empowered workforce and the right software tools, manufacturers can successfully deploy predictive maintenance strategies to increase uptime, reduce financial and environmental liabilities and improve operational safety.