Artificial intelligence-based tools provide remarkable insights leading to improvements in any domain that can be characterized with data.
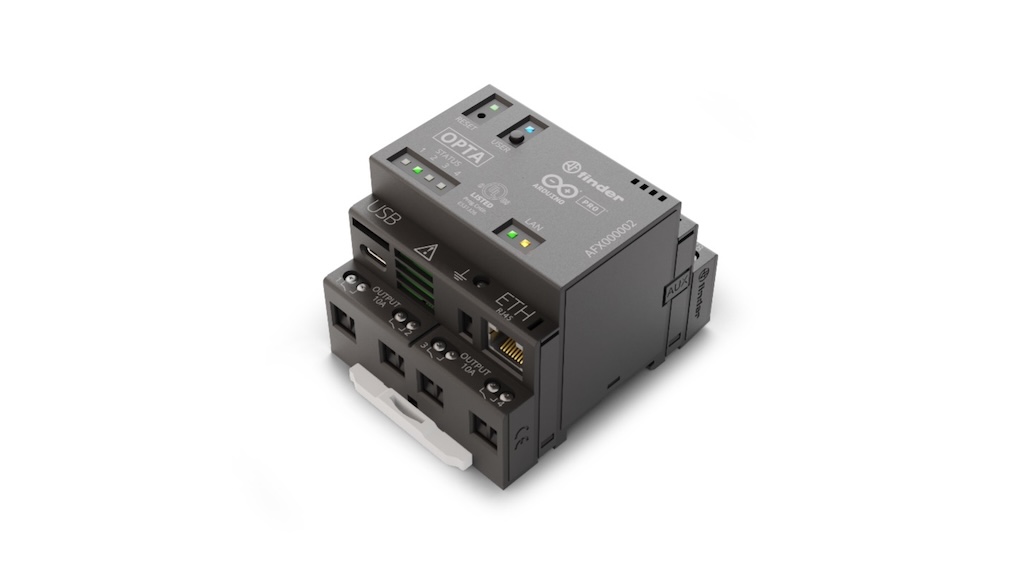
Learning Objectives
- Understand how data analytics can improve diagnostics, shifting manufacturers from reactive to predictive maintenance, minimizing downtime and repair costs.
- Learn effective strategies for integrating legacy systems without modifying them to retrieve valuable diagnostics data.
- Understand how system integrators are uniquely suited to resolve complex data integration challenges.
Data analytics insights
- Data analytics can help organizations understand disparate data sets to form a cohesive view of manufacturing floor performance.
- Machine learning can help manufacturers predict when problems could arise, saving downtime and money.
- Sensor placement plays a significant role in data analytics outcomes as collected data must accurately reflect operating conditions.
Data analytics can help organizations achieve remarkable results, and the data sets that fuel it are an integral part of this winning strategy. These artificial intelligence (AI)-based tools have become widely available to mathematically model, characterize and optimize nearly every part of a manufacturing operation.
Fueled by diverse data sets that describe the system, abundant in any manufacturing facility, the tools’ insights can show how to increase uptime, reduce costs and improve quality — all facets of overall equipment effectiveness (OEE). They do so by facilitating system diagnostics, predicting maintenance needs prior to equipment failure. While manufacturers commonly struggle with data accessibility and usability, investing in foundational steps to overcome this hurdle is essential for maintaining current and future competitiveness.
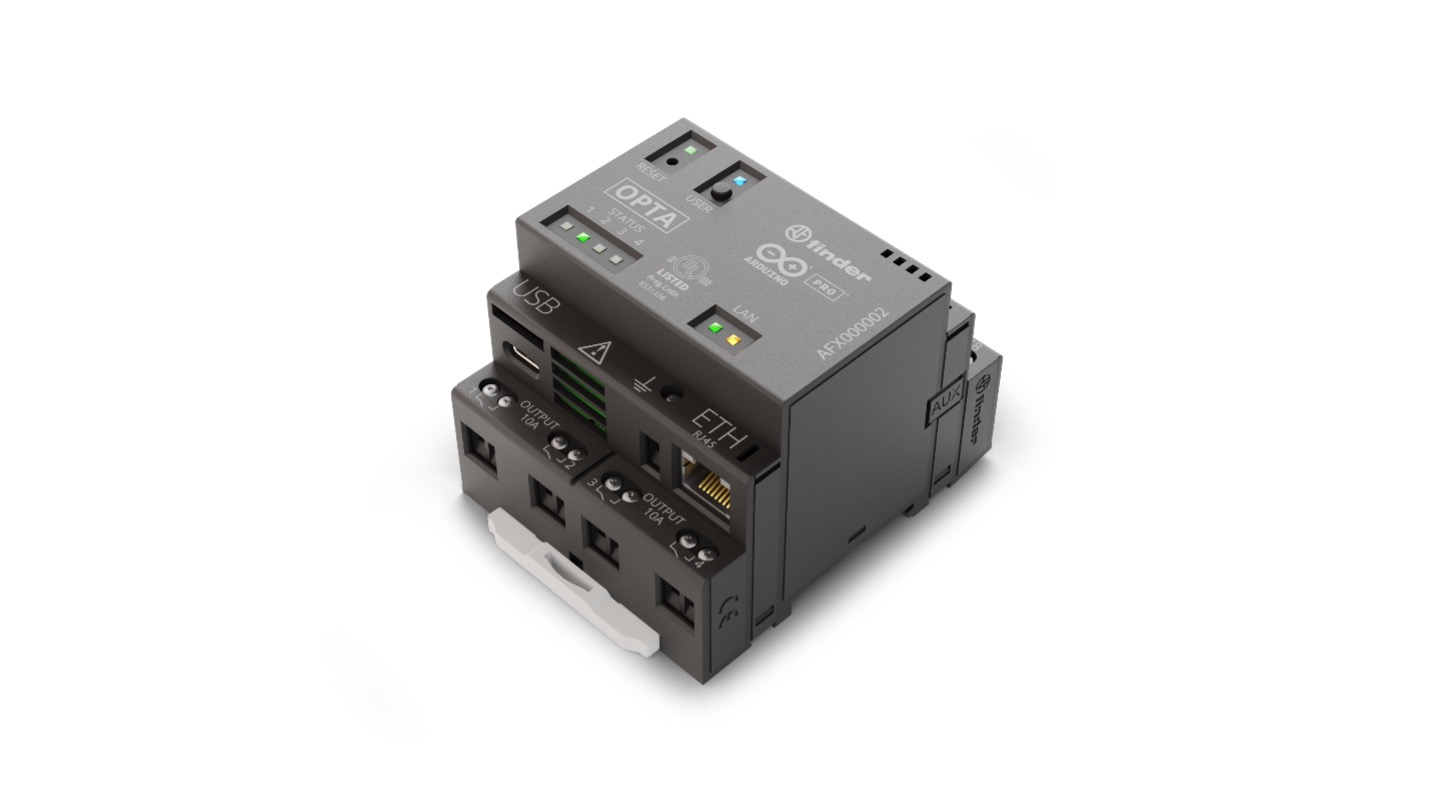
What is machine learning?
Machine learning, a subset of AI, employs vast data sets pooled from diverse parts of a system to train a model using sophisticated algorithms that mathematically describe its behavior. Once properly trained, the model can be used for insights in many different capacities, pulling together relationships among variables that may otherwise go unnoticed.
Data analytics is particularly well-suited to manufacturing because facilities already generate large amounts of data from various sources across the operation, including sensors, equipment performance metrics and operational efficiencies. When used effectively, machine learning algorithms can turn this data into actionable insights, leading to predictive maintenance, process optimization and better quality control.
Data analytics for root cause analysis of faults
A common vexing automation challenge faced by manufacturers involves prolonged downtime associated with unclear equipment failures. These incidents result in a cascade of stressful, reactive engineering and maintenance efforts and a significant loss of productivity.
Data analytics addresses this problem. By analyzing historical data, machine learning algorithms can identify the patterns that previously led to faults from past incidents. This capability not only speeds up diagnostics but also helps manufacturers transition from reactive to proactive maintenance, enabling them to predict issues and plan for their remediation prior to an expensive failure.
Consider a recent project Patti Engineering completed on behalf of a large industrial manufacturer that suffered a major press failure, halting production and ultimately costing the company more than $1 million in repairs. Had they known that the servo drive controlling the press was on the verge of failure, the issue could have been fixed for around $10,000.
Predictive maintenance strategies provide exactly this kind of foresight. Implementation involves adding sensors to the system, each providing ongoing data describing the machine’s overall health. In the case of the press’s servo drive, vibration sensors (accelerometers) positioned close to the bearing housings of the servo motor may have measured changes in vibration possibly stemming from bearing wear. Likewise, a temperature sensor positioned near the motor’s windings may have measured higher operating temperatures indicative of increased winding resistivity or insulation breakdown. Overheating faults can also occur when the power electronics within a drive begin to fail, so a sensor monitoring its ambient operating temperature may have noticed it increasing.
In conjunction with other relevant system data sets (for example, power consumption), the sensors’ data points can be analyzed and modeled by various machine learning-based platforms providing manufacturers with insights into anomaly behavior well ahead of a dramatic failure like that of the press. Cloud-based analytics platforms from Amazon Web Services (AWS) or Microsoft Azure can be used for this purpose. As a result, industrial maintenance transitions to a streamlined proactive approach that minimizes downtime, reduces repair costs and maximizes equipment lifespan.
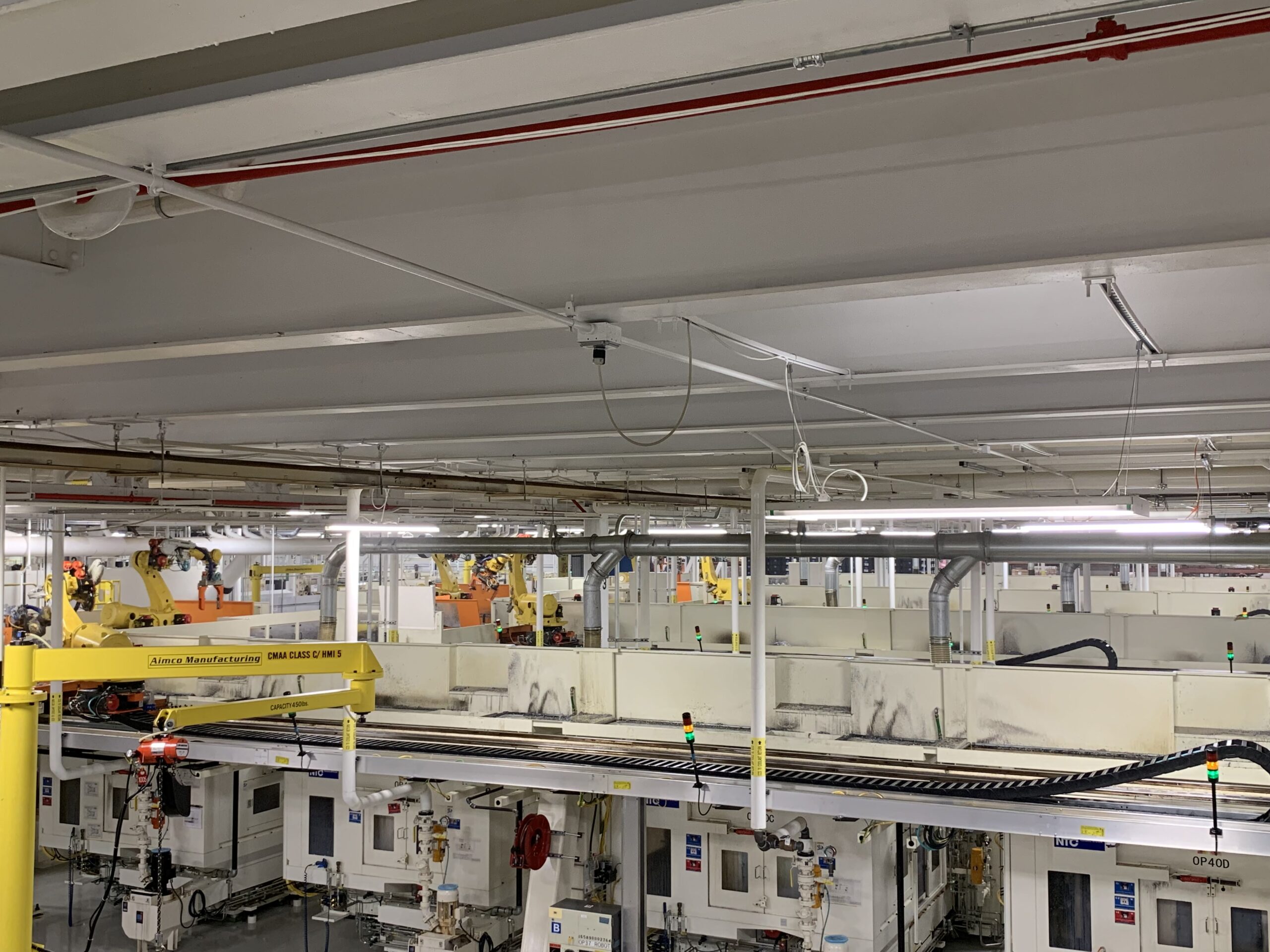
The role sensors play in data analytics
Patti Engineering’s approach to sensor integration is strategic with the goal of maximizing data accuracy while minimizing additional system complexity.
- It’s important to use the minimum number of sensors needed to characterize a system’s behavior, minimizing increases to the overall system’s complexity.
- Sensors should be integrated directly into the process, when possible, rather than positioning them in parallel. This approach often requires modifying the programmable logic controller program to route data to the selected cloud-based analytics platform. However, it ensures the collected data accurately reflects current operating conditions. In contrast, a sensor placed in parallel and not directly affecting production may fail without detection for extended periods, resulting in bad data and inaccurate models and insights.
Addressing disparate data sources with system integrators
While the benefits of advanced analytics-based applications are clear, they require access to clean, ready-to-use data that can accurately describe and therefore model the target system. Within the manufacturing industry, the hurdles to achieving this data-readiness are multi-faceted. Disparate and siloed data sources using different standards, protocols and formats with potentially differing reporting rates and accuracies must be addressed.
This challenge is uniquely suited to system integrators as they possess comprehensive knowledge of diverse vendors’ equipment and platforms and are skilled in creating interoperable systems.
Integrating legacy data systems
Another challenge to data integration within industrial manufacturing is the prevalence of legacy systems. Because they were not originally designed with current data needs in mind, the required data sets often do not natively exist within these systems. Compounding the problem, legacy systems are more prone to downtime events of unclear origin due to their age. Determining the cause of these events takes significant time, which takes a large toll on the manufacturer’s OEE.
To address this common problem, Patti Engineering partnered with Arduino. Arduino’s Opta facilitates the retrieval of valuable diagnostics data from older control systems without requiring their modification. Opta does what a legacy control system can’t do — collect, store, perform edge analysis and integrate data with modern aggregation platforms. It’s a tool that allows manufacturers to obtain better system diagnostics on legacy systems without immediately investing in a full controls upgrade.
Addressing manufacturing challenges with data analytics
AI-based simulation tools have the potential to deliver insights about a complex system’s operational efficiency that might otherwise be too difficult to discern. These tools not only facilitate root cause fault analysis but also foster predictive maintenance approaches that can prevent costly downtime. As a result, manufacturers that embrace AI-based tools will gain a competitive advantage by more effectively addressing a wide range of complex manufacturing challenges.
By relying on the expertise of system integrators for implementing foundational data integration strategies, including careful sensor use and other technologies that seamlessly interface with legacy systems, manufacturers can employ a winning strategy — consistently outperforming expectations, improving productivity and thriving amid resource constraints.