Subject matter experts and enterprise analytics increasingly allied
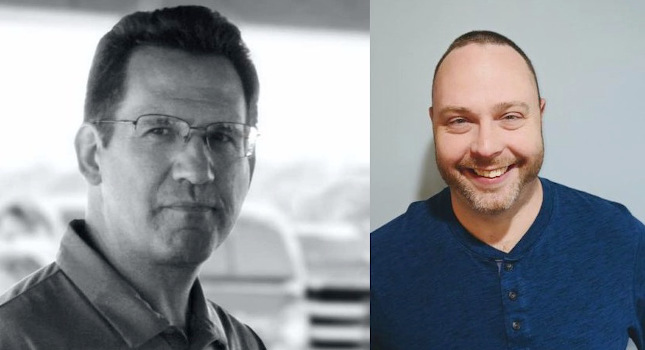
Not long ago, the Plant Engineering and Control Engineering brands of CFE Media & Technology presented a webinar on “What engineers must know about data science.”
Speakers on the webinar were Ed Kuzemchak, CTO and director of IIoT and embedded systems engineering and Sam Fahnestock, engineering manager and lead security engineer, both at Software Design Solutions (SDS), an Applied Visions Co.
Data scientists and data engineers are among the fastest growing occupations in the manufacturing industries, according to the U.S. Bureau of Labor Statistics. Yet relatively few of these data professionals are familiar with the unique parameters relevant to manufacturing and process-production environments.
One emerging tactic to fill this skills gap is that suppliers are introducing self-service applications, allowing engineers to take the initiative with minimum support from data engineers.
Evidence indicates engineers and technicians are “grasping the bull by the horn” by further educating themselves in the details of statistics and modeling. In this webinar, you’ll learn what they’re learning and how they’re learning it. To view the webinar in full, go to the Plant Engineering or Control Engineering websites.
At the end of the webinar, Sam and Ed answered questions from the audience. After its conclusion they put their heads together to answer the questions they didn’t get to. The questions and answers are found below.
Q: How much do today’s mechanical engineers need to know about data science?
A: The most productive modern engineering teams are moving in the direction of being cross-disciplined. The individuals on these teams often are experts in their normal roles but have some understanding and capability in other roles. Today’s mechanical engineers would benefit from some understanding of data science, allowing them to better understand and improve their products.
Q: Don’t most production industries share common characteristics when it comes to process control that make individual anomalies less important?
A: Many industries do share common characteristics in the types of data that may be collected, but each company will be unique in the specific return on Investment that it can make for a specific IoT project. That is why it is important to start small and measure the return. Also, engaging a third-party vendor that is familiar with industrial IoT can save a lot of time and money by bringing the best practices that are common to an industry.
Q: What industries have the capital to invest in upgrading facilities with emerging technologies?
A: Often, the best strategy for starting an Industrial IoT project is to retrofit existing equipment with low cost and off-the-shelf sensors. Industrial equipment has long service lifetimes, and this is a challenge that is somewhat unique to Industrial IoT compared to consumer IoT or even medical IoT. It is common for equipment to be in use for 20 or 30 years. Retrofitting existing equipment is more cost effective that replacing equipment with new IoT enabled equipment.
Q: What are the skills engineer’s need to master to take the data scientist’s role?
A: A basic understanding of mathematics and statistics is important to be able to take on the data scientist role within a team. There are many toolsets available that range from simple to complex; having the ability to use and understand some of these tools is also essential to taking on the role.
Q: You mentioned that the data scientist role can help the team better design the product. What type of analysis should they be doing to improve designs?
A: Pretty much any data analysis can provide insight to improving the design of the product. A good place to start would be analyzing what pieces of the system that users are using the most. By understanding the most used features, the team gains an understanding of the “backbone” of their application and can take purposeful steps to ensure those features are solid and high performing.
Q: We don’t have any data scientists at my company. How can we get started investing in data science?
A: Expanding a company’s capabilities is not a simple proposition. Often times bringing in outside help can help your company get moving on the correct path for long term success. A third party that understands, not only the ins and outs of data science, but the industry that you work in will guide you on what data to begin gathering and how to begin analyzing it.
Q: What would incremental releases of an IIoT project look like?
A: One of the most valuable lessons that I have learned in seeking to combine the agile mindset with IIoT projects is to take the time designing the hardware with future features in mind. It is often far easier to push out firmware updates to customers than it is to get them to switch out hardware. By adding a few extra sensors to the board up front, you will be able to deliver new features to customers through software.
Q: What are some of the external factors that influence the success of an Industrial IoT sensor project?
A:Industrial IoT is unique in that it combines several environmental factors that are challenging for sensors. Often, sensors must be battery powered, and batteries may need to last for a long period of time. Also, sensors are often used in harsh environments, so the selection of sensors needs to take that into account. Finally, wireless communication is often used, but it may be a challenge due to the interference caused by the amount of metal equipment nearby. One way to address the last concern is an early prototype with off-the-shelf equipment to assess the wireless communication feasibility.
Q: What data visualization and analytics package would you recommend?
A: At the start, we strongly recommend starting small and simple. Stay with what you are comfortable with – that might be something as ‘simple’ as MS Excel. As your IoT project matures, the analyses that you need will make themselves apparent and this will help guide you to the proper data visualization or analytics platform. The one positive use of seeing demonstrations of visualization and analytics platforms early is to help bridge the ‘imagination gap’ that can hold back project stakeholders.
Q: Can you expand on the “imagination gap?”
The imagination gap is the term that we use to describe when someone does not know that something is possible, therefore doesn’t even consider it when they look at options in the IoT landscape. Bridging this gap is important to help stakeholders see new opportunities and help define roadmaps of where the project might go in the future.
Q: Are there legal issues involved with collecting data for machine learning and data science purposes?
A: As with everything legal, we recommend consulting with your company’s legal team to find out the specifics of what you can and cannot collect. This type of data collection often requires an agreement from the end customer, allowing the collection of usage data.
Q: How can we get started on an IoT project?
A: Gather the stakeholders that were discussed, and include a IoT engineering firm in that early meeting that can bring best practices, so you do not have to repeat others mistakes, and also help lay out an agile, phased roadmap that has incremental wins along the way.