Capturing the full complexity of tribological contact requires careful integration of disparate phenomena.
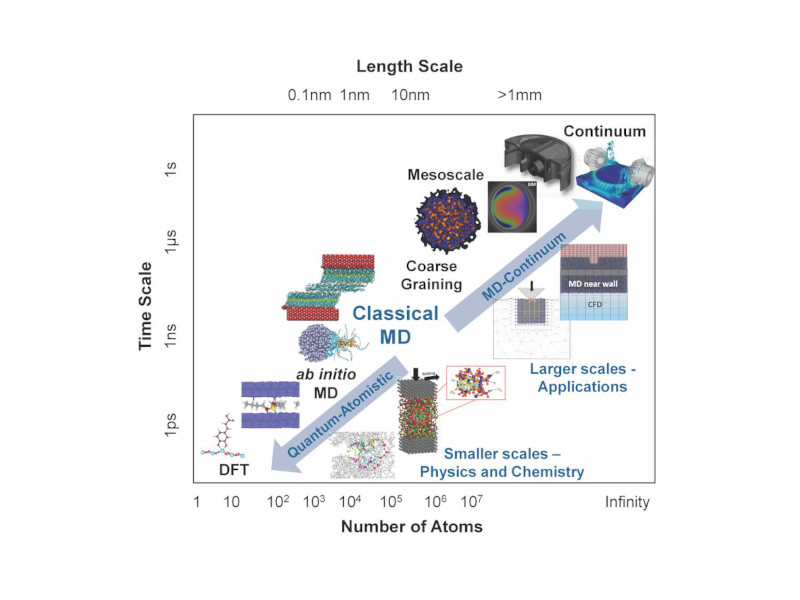
Learning Objectives
- Understand why modeling is important for tribology.
- Learn about future tribological models and what they can do for tribology and lubrication.
Tribology insights
- Historically limited by senses, modern research employs computer modeling to explore phenomena, transforming engineering by simulating intricate tribological interactions previously inaccessible.
- From complex multiscale dynamics to computational limitations, tribological modeling demands nuanced approaches, integrating diverse disciplines and technologies for accurate predictions and design improvements.
For most of history, humans who wanted to understand the physical world were limited to what they could explore with their own senses. Although our senses are vastly extended by powerful scientific instruments, the idea of experimentation is essentially the same as it has been for millennia. Today, however, researchers also have a completely different mode of exploration: computer modeling. By representing a physical system mathematically through a computer program, researchers can simulate phenomena that would be impossible to observe even with the most sensitive instruments.
Modeling is important in tribology because everything interesting is happening on the tiny, tiny patches where surfaces are in contact. (Even apparently flat surfaces are in contact only on a small area.) These contact patches are measured in micrometers or even nanometers. What happens in these tiny patches dominates the behavior of the entire system, whether that be blood cells sliding along blood vessels or bearings turning in the gearbox of a huge, 10-megawatt wind turbine. The problem is, in most cases, we can’t observe the contact patches directly.
Understanding how contacts work is important because tribological interfaces are central to the design of virtually every product and manufacturing technology. They are hard to observe, however, so engineers have traditionally had to spend large amounts of time and money on physical prototyping to achieve correct performance. Today, by using models of contact phenomena, engineers can replace physical prototyping with simulated performance testing.
There’s a catch, though. Although tribological behavior begins within a small contact domain, what happens in that domain is influenced by what’s happening in its vicinity, for example, how well the lubricant flows or whether the interface is externally heated.
The broad scientific challenge is both conceptual and empirical. A holistic understanding of interfaces requires knowledge of their shape, structure and chemistry from the atomic to the macroscale, as well as their dynamic evolution under a range of often extreme operational conditions—temperature, pressure, shear, chemical reactivity, strain and so on,” said STLE member professor Daniele Dini, head of the tribology group in the Department of Mechanical Engineering, Imperial College London.
This constellation of influences means that contact modeling is considered a “multiphysics” problem; that is, significantly different governing equations must be solved. This problem is compounded by the fact that the field of tribology spans the most disparate of applications, from biology to aerospace. Thus, what is recognized as an appropriate modeling solution in one area cannot be easily translated to another area. Furthermore, very different approaches may be needed when treating, for example, frictional interactions in soft tissues at a cellular level (which requires biological as well and engineering knowledge) versus in bearings used in a wind turbine in the presence of harsh environmental conditions.
[subhead] Why modeling even simple contact is complex
Michael Watson, postdoctoral researcher at the University of Sheffield, gives some examples of the factors that make contact modeling so difficult:
“For the simplest example of frictionless, dry, elastic contact, while the contact patch might spread over millimeters, individual asperities [rough areas] might be contacting on a micrometer length scale. Because of that, we need to discretize the relatively large contact into very small points. This puts the solution out of reach of normal finite element solvers and means we have to use a trick, namely, pretending that the body is a flat half space [extending infinitely from a flat boundary], to solve the stress equations. Sometimes this works well, but sometimes it doesn’t. In particular, any phenomenon that depends on the true 3D shape of the surface, such as a spiky asperity getting flattened, needs to be modeled by a fully 3D solution to the stress equations, but such a solution is out of reach for current technology.
“Another problem we often face is pushing materials beyond their linear region. For example, most dry contacts involve some sort of plastic deformation, but even for the simplified flat case, deformation is extremely costly to calculate. Also, the flat-case simplification often won’t capture the sort of behavior most people think about when talking about plastic deformation in a contact (e.g., ploughing or flattening of asperities).”
Phenomena at a tribological contact
“Tribological contact is inherently one of the most complex engineering scenarios that modelers face,” said Daniele Dini, head of the tribology group, Department of Mechanical Engineering, Imperial College London. Among the phenomena that must be considered include:
-
Origin of friction
-
Surface roughness
-
Evolution of tribofilms (solid surface films)
-
Evolution of material microstructure
-
Evolution of damage near surfaces
-
Deformation
-
For lubricated contacts: Fluid rheology
-
Changes in fluid film behavior.
If such broader influences aren’t considered, simulation results may be flawed—which could prove costly. Thus, a key challenge in modeling a tribological contact is the need to account for phenomena that occur at vastly different orders of magnitude in both size and time and that involve very different physics, all within the same model—an approach known as multiscale modeling.
The multiscale problem
“It’s best to see “modeling in tribology” as a continuum from trying to predict the friction coefficient of some simple system (think diamonds in a vacuum) from first principles to using a statistical model to describe the results of a big, dirty experiment. In the middle are mixed models that use first principles to describe stresses, or empirical models based on experimental data,” said Michael Watson, postdoctoral researcher at the University of Sheffield. One approach to solving the multiscale problem is to connect models designed for different scales.
In a comprehensive technical review of contact modeling, Vakis and colleagues describe multiscale modeling as: “A technique in which two (or more) different models related to different [scales] (or different matter descriptions) interact, i.e., exchange data, in a way that enhances the information that can be obtained about the model phenomenon.”
Figure 1 illustrates some modeling techniques that are used to study contacts. Various contact modeling solutions promising to overcome the scale issue are already available, although they tend to be optimized for solving specific problems. They span a vast range in complexity, cost and functionality and have been used in many practical applications to solve real world problems, for example to study friction in train wheels.
![Figure 1: Tribological systems are simulated with different techniques at different scales. An area of current interest is linking molecular dynamics (MD) simulations to smaller scales through quantum mechanical descriptions (ab initio or first principles MD models and density functional theory [DFT] models) and to larger scales through continuum models (including computational fluid dynamics [CFD] models).](https://www.plantengineering.com/wp-content/uploads/2025/02/PLE2406_MAG_LUBE_STLE_TRIBOLOGY_CONTACT_Figure1.jpeg)
First, the required computation time may be impractical (or outright impossible). According to Mahdi Mohammadpour, associate professor/senior lecturer, Loughborough University, UK, and founder of TriboDENS, “The multi-scale problem has been tackled by incorporating different simulation assumptions or computational accelerators (e.g., multigrid modeling). These solutions have resulted in computational enablers in tribology, but a reasonably realistic simulation still requires high-performance computing facilities. If multiphysics complexity is added (e.g., tribo-dynamics in rotating machinery), the solution may be completely unfeasible.”
Second, although the compromises or assumptions mentioned by Mohammadpour make computation practical, they can introduce new problems. When models are later connected, assumptions made at one scale may, when used beyond their original scope, distort the calculations at another scale.
Use with caution
Although multiscale models are being used successfully in engineering applications, good physical judgment also is required. A simulation may spit out a result that looks useful, but such results cannot be used and trusted blindly; they need to be evaluated and interpreted by experts.
As Vakis and coauthors pointed out, “An important question in multiscale modeling is the following: how to identify which spatial and temporal scales and mechanisms are relevant for understanding the phenomena to be modeled.”
They note a “simple recipe” is to introduce additional scales, not only in terms of dimensions but also in the type of computational approach.
However, they pinpoint a key weakness. “This simple recipe can often be ineffective as it depends on the ability of the ‘user’ to add the right details at the right scale, and may lead to the neglect of important information flow across the scales.”
Any engineer or researcher using such models should, therefore, think carefully about the cumulative impact of assumptions made at various scales.
Integration into design
Such models have given us a better understanding of the interactions that govern the response of engineering systems, but Dini identified some pitfalls for designers:
“These models are often problem-specific and fail to enable full integration in design strategies. Furthermore, they often consider only snapshots. We need a simulation strategy and a platform that allows engineers a convenient way to put tribology at the center of the design strategy. There is a need for flexible, predictive models of interfaces that describe their operando evolution.”
Validation
Watson raised another concern: “Each point along the scale continuum has its place and its own specific challenges, but one challenge that hits every point but that I rarely see discussed is validation.”
Because “first principles” models (e.g., molecular dynamics simulations or Hertzian contact mechanics) aren’t expected to get the right answer the first time, they can’t be fully depended on in any situation.
“That’s why people commonly add empirical models that they think capture the physics at play, but they typically stop tweaking the model when it fits the data on hand,” Watson said.
As a result, the correlation between models and the real world appears better than is warranted by the underlying representation.
“In essence, a model is a theory of how something works, and, as a theory, its test should be its predictive power, not how well it fits to the data available when it was made,” Watson said.
A further difficulty, according to Watson, is these models oten are not fully released to the research community, or they turn out to have issues when tested outside the original parameters, so comparing reported results remains difficult. Still, there are notable exceptions. Watson cited open source, cutting-edge codes such as Tamaas and said some lubrication models are approaching good estimates of friction from first principles, even for elastohydrodynamic lubrication.
Asking the right question
The issue is sometimes more fundamental, as noted by Klaus Six, key researcher, Virtual Vehicle Research Center, Graz, Austria.
“In doing a lot of reviewing work for journals and for doctoral theses, one of the main problems I see is that people start to work without defining a clear research question. First of all, you need to make clear what phenomenon you want to understand or predict, and then start thinking about modeling and testing. I very often have the feeling that people work the other way around,” Six said.
Just using a complex model that takes a lot of calculation time does not guarantee more accurate results.
“If the model is not able to describe relevant physical phenomena, it’s useless. First describe clearly what you want to predict, then think carefully about what the main physical effects are, and only then start thinking about what modeling approach is right,” Six said.
Three future tribological models
Progress on this complex problem will require developments along several avenues, including changing how models are created and shared, exploring how modeling interacts with experimentation and integrating new computational tools.
1. Democratization and open source
Mohammadpour pointed to a technical and social challenge:
“In my view, the greatest challenge of computational tribology is lack of democratization in this field. Computational methods often require highly skilled tribologists to be successfully deployed. It is widely accepted that a tribology simulation will never be as accessible as a structural finite element analysis, but the current level of accessiblity is not good enough. There are examples of attempts to resolve this problem to allow engineers even at the graduate level to perform tribological simulations.”
One such solution is a free Python package for tribology called Slippy developed at the University of Sheffield and the University of Leeds. The code provides a framework for a common approach to equations across multiple scales, according to STLE member Tom Slatter, professor, department of mechanical engineering, the University of Sheffield.
Slippy makes training in contact modeling more accessible, even to undergraduates, because the user doesn’t need to start with actual surface data. A student can generate a random surface and then explore it with other parts of the Slippy package.
“It’s an application that’s meant to help people just getting into tribology,” Slatter said.
While it is important to improve access to training in tribological modeling, it also is important to improve the accessibility of both the models themselves and the data that are generated from the models or used to validate their implementation and verify their predictive capabilities.
Watson said, “I believe a large part of future modeling must be open source. I have implemented many models from papers that claim good results, only to be disappointed either by the performance of the model itself or by the paper missing out hidden factors or nontrivial solution details. Normal peer review is not enough to catch small mathematical typos, but these can render a paper almost unusable. If the modeling code for a paper exists and runs, we as a community should require its release as the true object of peer review.”
2. Models and simulations replacing experiments
For some purposes, models and simulations are good enough to replace physical testing. This success has led some to speculate that experiments will soon become largely unnecessary. Of course, models are only as accurate as their inputs, so data will still be required for material, lubricant, surface and so on. Therefore, experiment in this sense never goes away. However, “if by experiment we refer to a physical test for validation of the design or simulation,” Mohammadpour said, “this need will ultimately be eliminated in many subjects, especially those which are well established. This is already happening in big marine systems, for example, as the unique engines and transmissions of these systems do not give opportunity for prototyping. Therefore, the developers rely on simulation and calculations, which work surprisingly well. The problems may happen when new physics are introduced. An example is the electric field present in modern electrified powertrains, which leads to different behavior of the tribofilm and the lubricant and to novel failure modes.”
Watson also believed this speculation is overstated: “I don’t believe that simulation will fully replace experiments in my lifetime, but I do think we will see an increase in models driving design and informing experiments.”
Dini agreed, saying, “Given the complexity of the mechanisms involved in tribological interfaces and the variety of applications, it will be probably impossible to ever replace experimental testing completely. What I believe will happen is that computational models will be used for investigations that are extremely difficult to perform, especially at the smallest of scales, but there will always be a need for targeted experiments to complement and validate models, especially when new solutions and products are developed.”
Data also will continue to be needed at the beginning of the modeling process as well, Dini said, as the foundation for developing “digital twins”—paired real and virtual processes or components that are used iteratively to improve the performance of the other.
Advances in experimental and characterization techniques can change the equation too, Dini notes. An example is the use of multiple techniques to probe a single sample, whether at in-house labs or at synchrotron X-ray facilities; such techniques can provide data on previously inaccessible aspects of interfacial behavior.
3. Artificial intelligence and other computational strategies
New tools are emerging on the computational side, too. “The next step will be significant enhancement of simulation speed using artificial intelligence. This will break barriers and allow coupling of multiphysics and multiscale simulations that is currently impossible,” Mohammadpour said.
Dini agreed. “Machine learning can speed up the integration between models and experimental data as well as contribute to the development of new disruptive data-driven modeling tools.”
Watson also agreed. His view is speed can be achieved by treating models more statistically in general, both with machine learning and with other statistical approaches.
“In my opinion, the future of tribology modeling is embracing statistical solutions to questions we would traditionally answer numerically. Great progress has been made in graphics simulation problems by implementing machine learning models for a special formulation of the Navier-Stokes equations. These models allow the equations to be approximately solved extremely quickly. Such models would unlock solutions to a host of truly 3D behaviors that remain out of reach of traditional solvers,” Watson said.
A roadmap for tribological progress
According to Dini, taking tribological modeling and simulations to the next level will require progress in three areas. Researchers and engineers need to:
-
Embed various machine learning and data-handling tools into all stages of the process of designing new tribological solutions. Tasks for such tools could range from the analysis of experimental data to autonomous design of molecules and materials.
-
Integrate the traditionally distinct stages of modeling and experiment. With the two more tightly coupled, synergies will be found between information available across scales.
-
Develop accurate virtual replicas of experiments, components and engineering systems. Such replicas will support end-to-end optimization of tribological interfaces with respect to key performance targets (including time-evolution, efficiency and long-term reliability).
Understanding a complex problem like tribological contact will require multifaceted solutions. As shiny new modeling strategies arrive and computing facilities become ever more powerful, the challenge for modelers and engineers is to remember to return, over and over, to the fundamental processes occurring at interfaces.
Reprinted with permission from the December 2023 issue of TLT, the official monthly magazine of the Society of Tribologists and Lubrication Engineers, a CFE Media and Technology content partner.
CAPTIONS
Figure 1: Tribological systems are simulated with different techniques at different scales. An area of current interest is linking molecular dynamics (MD) simulations to smaller scales through quantum mechanical descriptions (ab initio or first principles MD models and density functional theory [DFT] models) and to larger scales through continuum models (including computational fluid dynamics [CFD] models). Courtesy: Daniele Dini/STLE
REFERENCES
- “An elastic half space is an elastic material that extends infinitely in all directions including depth with the surface at the top considered as the boundary,” in S.-V. Kontomaris and A. Malamou (2020), “Small oscillations of a rigid sphere on an elastic half space: A theoretical analysis,” European Journal of Physics, 41, 055004, https://doi.org/10.1088/1361-6404/ab9a0a.
- Vakis, A.I., Yastrebov, V.A., Scheibert, J., et al. (2018), “Modeling and simulation in tribology across scales: An overview,” Tribolology International, 125, pp. 169-199, https://doi.org/10.1016/j.triboint.2018.02.005.
- Fukagai, S., Watson, M., Brunskill, H.P., Hunter, A.K., Marshall, M.B. and Lewis, R. (2021), “In situ evaluation of contact stiffness in a slip interface with different roughness conditions using ultrasound reflectometry,” Proceedings of the Royal Society A: Mathematical, Physical, and Engineering Sciences, 477, 20210442, https://doi.org/10.1098/rspa.2021.0442.
- Ewen, J.P., Heyes, D.M. and Dini, D. (2018), Advances in nonequilibrium molecular dynamics simulations of lubricants and additives, Friction, 6, pp. 349-386, https://doi.org/10.1007/s40544-018-0207-9.
- Tamaas — A High-performance Library for Periodic Rough Surface Contact, hosted by École Polytechnique Fédérale de Lausanne, Laboratoire de Simulation en Mécanique des Solides, https://tamaas.readthedocs.io/en/latest/.
- Slippy: A Python Package for Tribologists, developed by the University of Sheffield and the University of Leeds under the “Friction: The Tribology Enigma” Programme Grant, funded by the UK Engineering and Physical Sciences Research Council (EP/R001766/1), https://friction.org.uk/slippy-software/.
- Walker, J., Questa, H., Raman, A., Ahmed, M., Mohammadpour, M., Bewsher. S.R. and Offner, G. (2023), “Application of tribological artificial neural networks in machine elements,” Tribology Letters, 71, 3, https://doi.org/10.1007/s11249-022-01673-5.