Generative artificial intelligence (AI) is transforming predictive maintenance by improving failure predictions, optimizing workflows and creating a self-improving system that enhances asset reliability and efficiency.
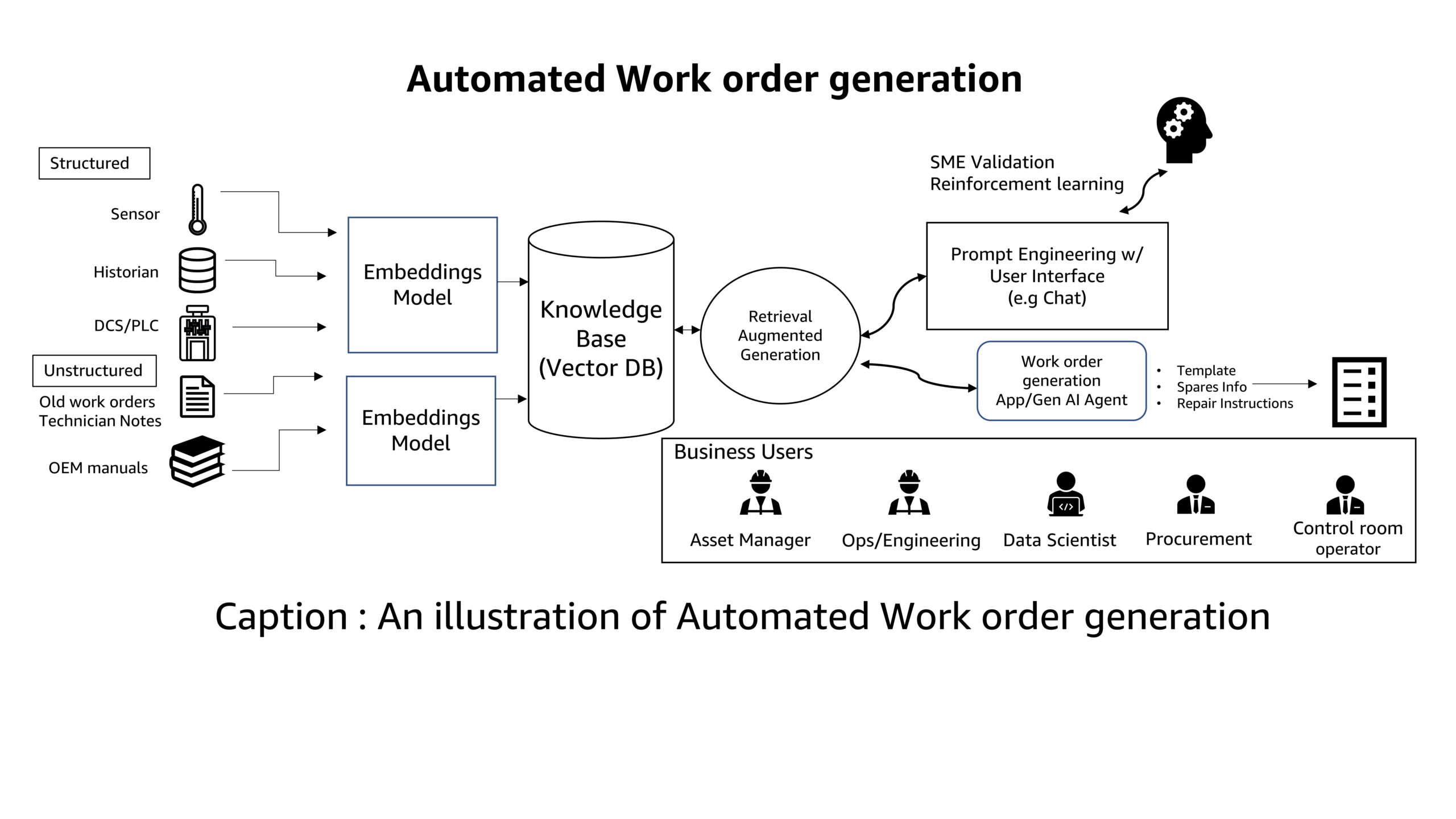
Learning Objectives
- Understand the role of generative artificial intelligence (AI) in predictive maintenance.
- Learn a six-phase framework for AI-powered maintenance.
- Recognize the benefits, challenges and mitigation of risks of AI-powered maintenance.
AI insights
- This article outlines a six-phase framework that integrates AI-driven analytics with human expertise to enable a proactive, cost-effective maintenance strategy.
- It dives deep into the six-phase framework for integrating AI into predictive maintenance, detailing required data inputs, infrastructure, tools and expected outcomes at each stage.
- It also addresses governance strategies and best practices to ensure AI-driven maintenance is secure, transparent and scalable, empowering human expertise rather than replacing it.
Generative artificial intelligence (AI) is transforming industrial maintenance by identifying failures before they disrupt production, reducing downtime and optimizing asset performance. Traditional maintenance approaches, whether reactive, preventive or early predictive, often lack precision, leading to unnecessary repairs, missed failures and increased costs.
As a result, these methods require significant human intervention, forcing engineers and technicians to perform repetitive, non-value-added tasks, such as manual data analysis, routine inspections and unnecessary servicing. Generative AI eliminates these inefficiencies by automating diagnostics, analyzing vast datasets in real time and continuously refining predictive insights, enabling maintenance teams to focus on higher-value decision-making.
However, AI adoption introduces challenges such as data integrity, model reliability, cybersecurity risks and workforce adaptation.
The evolution of maintenance strategies
Maintenance strategies have evolved with industrial complexity, each step building the foundation for the next. Reactive maintenance, the earliest approach, was simple but costly, leading to unplanned downtime and operational disruptions. Preventive maintenance introduced scheduled servicing to mitigate failures but often resulted in unnecessary repairs and inefficiencies because servicing was performed regardless of asset condition.
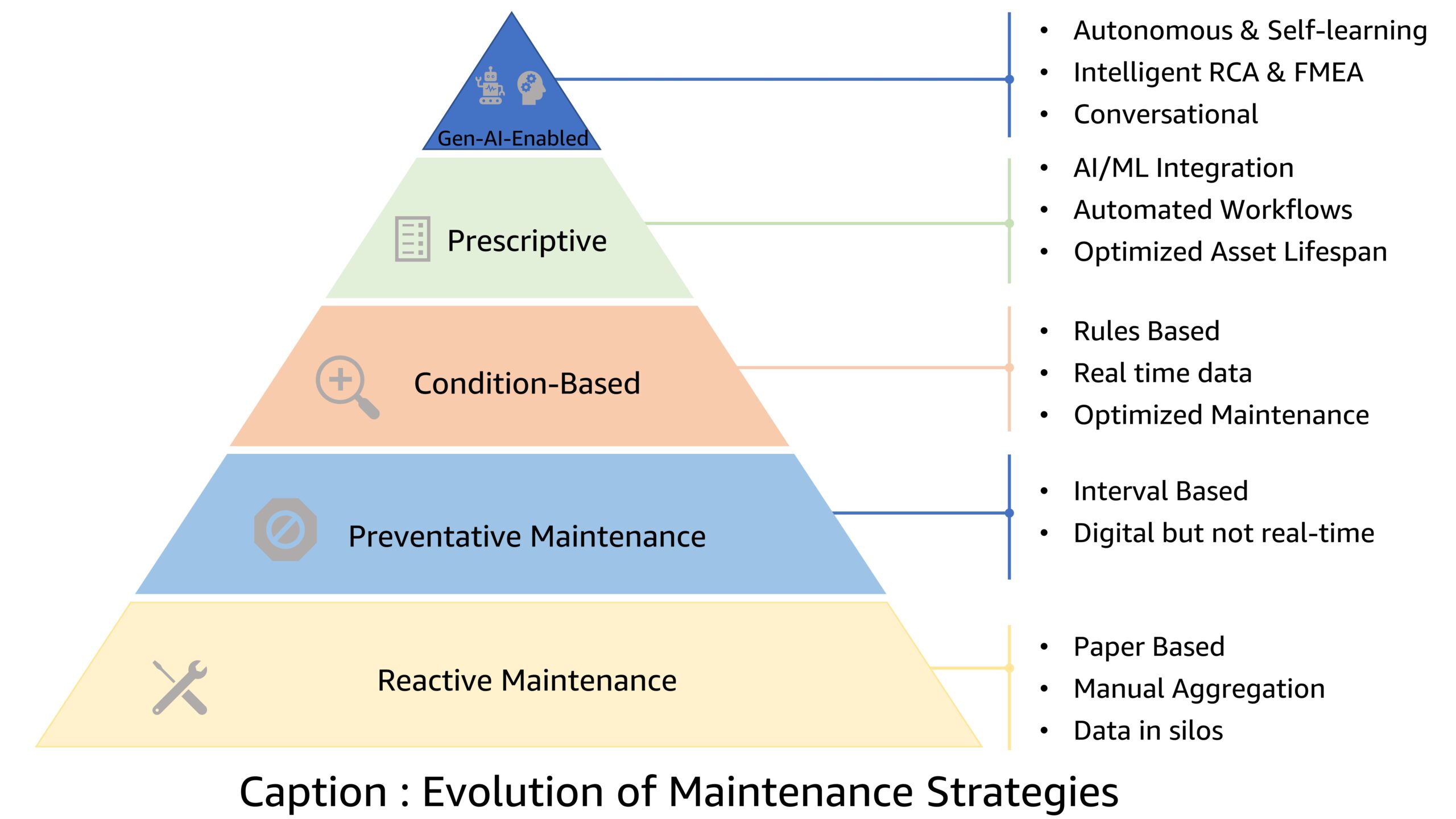
Condition-based maintenance leveraged sensor data for real-time monitoring, allowing interventions based on performance deviations rather than fixed schedules. However, static thresholds limit its ability to anticipate complex failure patterns. Prescriptive maintenance, enabled by AI, introduced predictive insights by analyzing historical and live data to optimize interventions and reduce downtime.
Now, generative AI is revolutionizing maintenance by creating an autonomous, self-learning system that continuously analyzes vast datasets (structured and unstructured), detects hidden patterns and refines predictions dynamically. Unlike previous approaches, generative AI adapts in real time, optimizing maintenance execution and aligning with operational goals. This shift from reactive to AI-driven intelligence ensures maximum reliability, cost efficiency and asset longevity.
The rise of generative AI in industrial maintenance
AI has evolved from rule-based automation to machine learning models capable of analyzing vast datasets and making predictions. Early AI relied on structured logic, but as computing advanced, machine learning enabled AI to identify patterns and forecast failures, making it an essential tool in industrial maintenance.
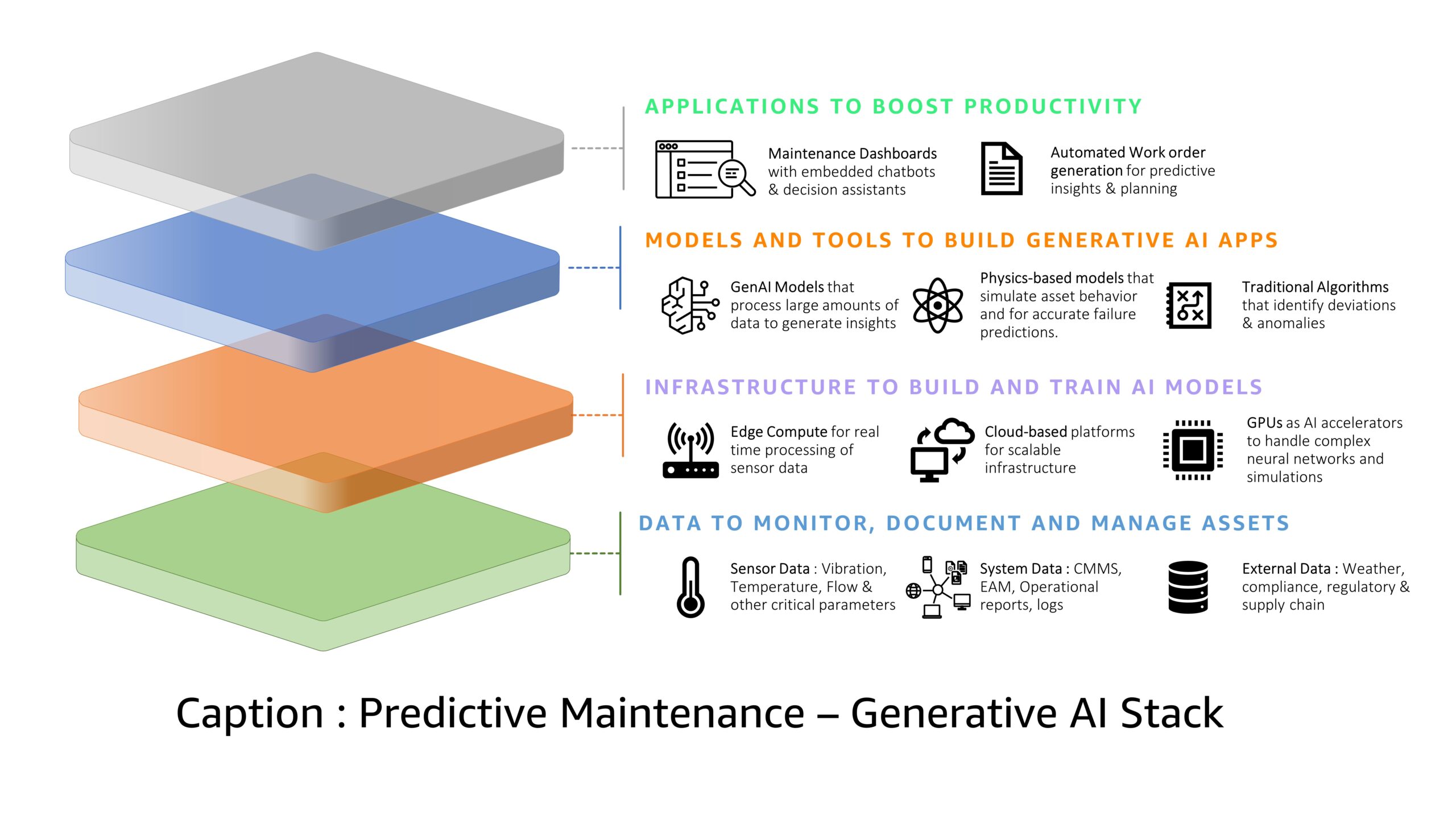
Generative AI represents the next leap, moving beyond pattern recognition to generate insights and dynamically refine strategies. Unlike traditional AI, which relies heavily on structured data, generative AI can process and combine structured and unstructured data, sensor readings, maintenance logs, operational reports and external factors, providing a more comprehensive understanding of industrial asset health. McKinsey & Co. estimates its economic potential at $2.6 trillion to $4.4 trillion annually, highlighting its transformative impact across industries.
For asset-heavy industries, generative AI unlocks new levels of efficiency by balancing cost, performance and risk in asset management. Industrial maintenance has long relied on predictive models, but these often operate within rigid frameworks. Generative AI continuously adjusts strategies based on real-time sensor data, historical trends and contextual factors, allowing for more responsive and cost-effective maintenance.
However, to fully leverage generative AI, organizations need a strong data foundation. High-quality, real-time inputs from sensors, computerized maintenance management systems (CMMS), asset monitoring tools and work order systems are critical. Without these, even the most advanced AI struggles to provide meaningful insights. By integrating these foundational data layers, companies ensure AI has the necessary context to optimize maintenance workflows and maximize asset value.
With a well-established digital infrastructure, generative AI can redefine industrial maintenance, enabling companies to move beyond static schedules and toward a more intelligent, adaptive approach. The convergence of AI, internet of things (IoT) and advanced analytics is no longer a vision for the future; it is the next phase of industrial efficiency.
A six-phase generative AI-infused framework
A predictive maintenance framework can be broken into six phases:
Phase 1: Asset criticality assessment and hierarchies
Traditional machine prioritization for predictive maintenance relies on manual analysis, where factory personnel compile failure data and maintenance managers use their experience to determine priorities. While this approach has been effective, it is time consuming, prone to inconsistencies and often constrained by subjective interpretation, leading to inefficiencies and misallocated resources.
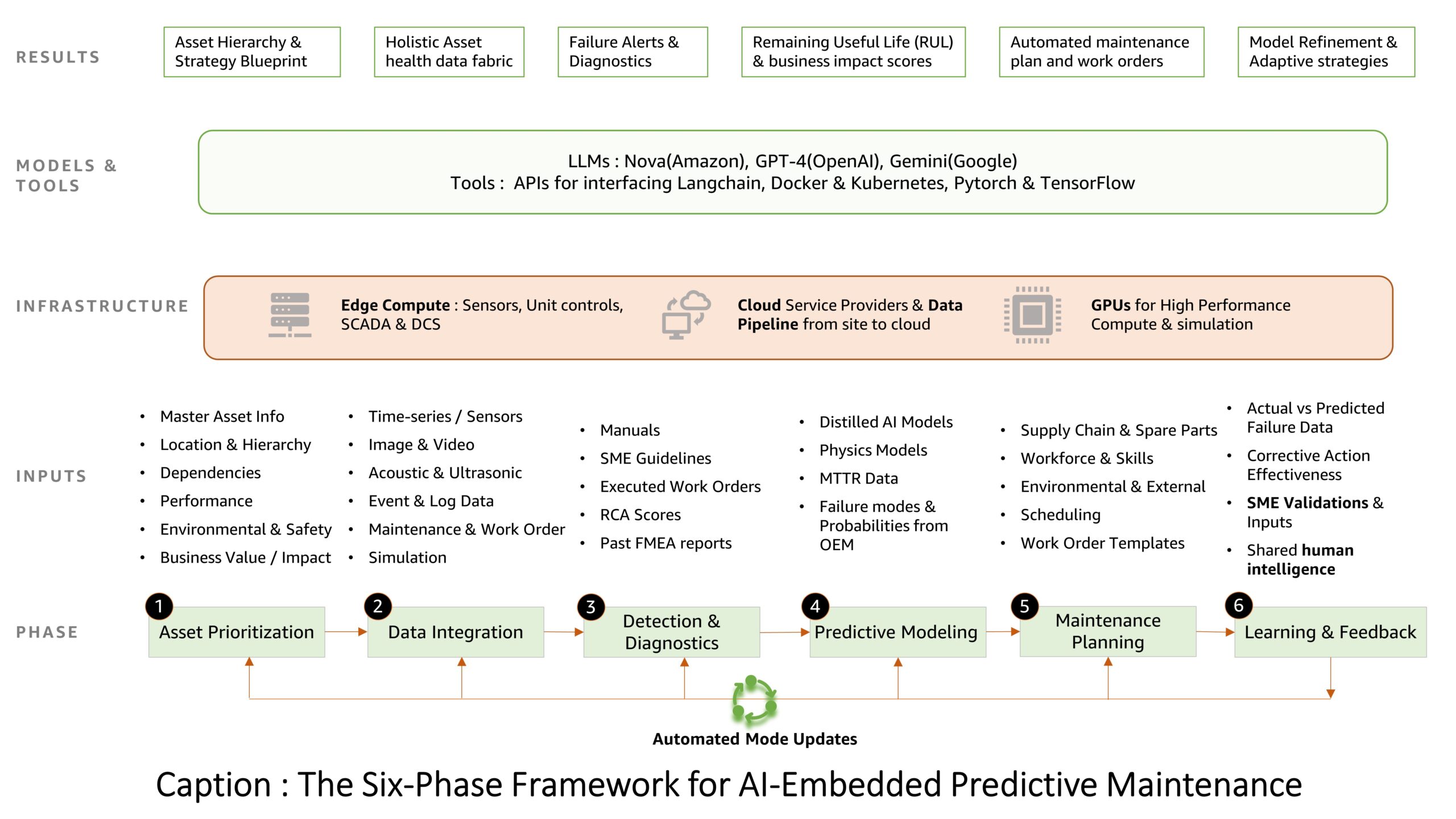
Generative AI transforms this process by automating analysis and dynamically prioritizing machines based on real time and historical data. By integrating structured and unstructured data such as sensor readings, maintenance logs and operational reports, AI can identify patterns, detect anomalies and continuously refine maintenance strategies. This eliminates reliance on manual reviews and ensures data driven decision making.
To maximize generative AI’s potential, organizations need a robust data infrastructure that enables semantic searches and contextual analysis. With AI driven machine prioritization, manufacturers can reduce downtime, optimize maintenance efforts and improve operational efficiency, unlocking a new level of proactive and intelligent asset management.
Phase 2: Sensor integration and data acquisition
A robust data collection infrastructure is the backbone of real time monitoring and AI driven predictive maintenance. However, many industrial assets still lack sensor-based monitoring. In industries like downstream oil and gas, only critical assets such as compressors, turbines and high-pressure pumps are typically equipped with sensors, while most other equipment follows traditional time-based maintenance schedules. This gap in data collection has historically been due to high sensor costs and infrastructure limitations.
With the rapid decline in sensor and cloud service costs, widespread sensor deployment is more feasible than ever. However, indiscriminately placing sensors on all equipment is neither practical nor cost-effective. Instead, sensor deployment should be guided by the asset criticality assessment from Phase 1, ensuring that only high-risk, high-value assets are continuously monitored. By strategically integrating IoT sensors, real time data pipelines and edge or cloud processing, organizations can create a scalable, efficient and intelligent maintenance system that maximizes reliability while keeping costs under control.
Phase 3: Anomaly detection and diagnostics
Anomaly detection and diagnostics are crucial for anticipating failures before they escalate. AI powered anomaly detection identifies deviations from expected performance, allowing maintenance teams to act before failures lead to costly downtime. However, diagnosing faults requires more than analyzing individual assets in isolation. Effective detection must correlate asset level performance with broader process conditions.
Traditional systems often miss complex failure patterns by relying on static thresholds. Motor overheating, for instance, might signal an issue, but generative AI can analyze sensor data, maintenance logs and process variables to reveal deeper causes. It may determine that fluid viscosity changes in a pump, not the motor itself, are causing excessive load and heat.
Beyond simple anomaly detection, AI powered root cause analysis identifies failure patterns, while fault classification ranks risks by severity. By leveraging a unified AI driven view of equipment and processes, organizations can shift from reactive detection to intelligent diagnostics, ensuring efficient maintenance and minimal downtime.
Phase 4: Predictive modeling and remaining useful life estimation
Generative AI weaves together sensor data, maintenance logs, process parameters and environmental factors into complex relationships that enhance failure prediction. This holistic modeling allows teams to forecast failures and remaining useful life (RUL) with greater precision. When real failure data is scarce, generative algorithms simulate realistic failure scenarios to enrich training data. These virtual examples boost prediction accuracy when actual incidents are rare.
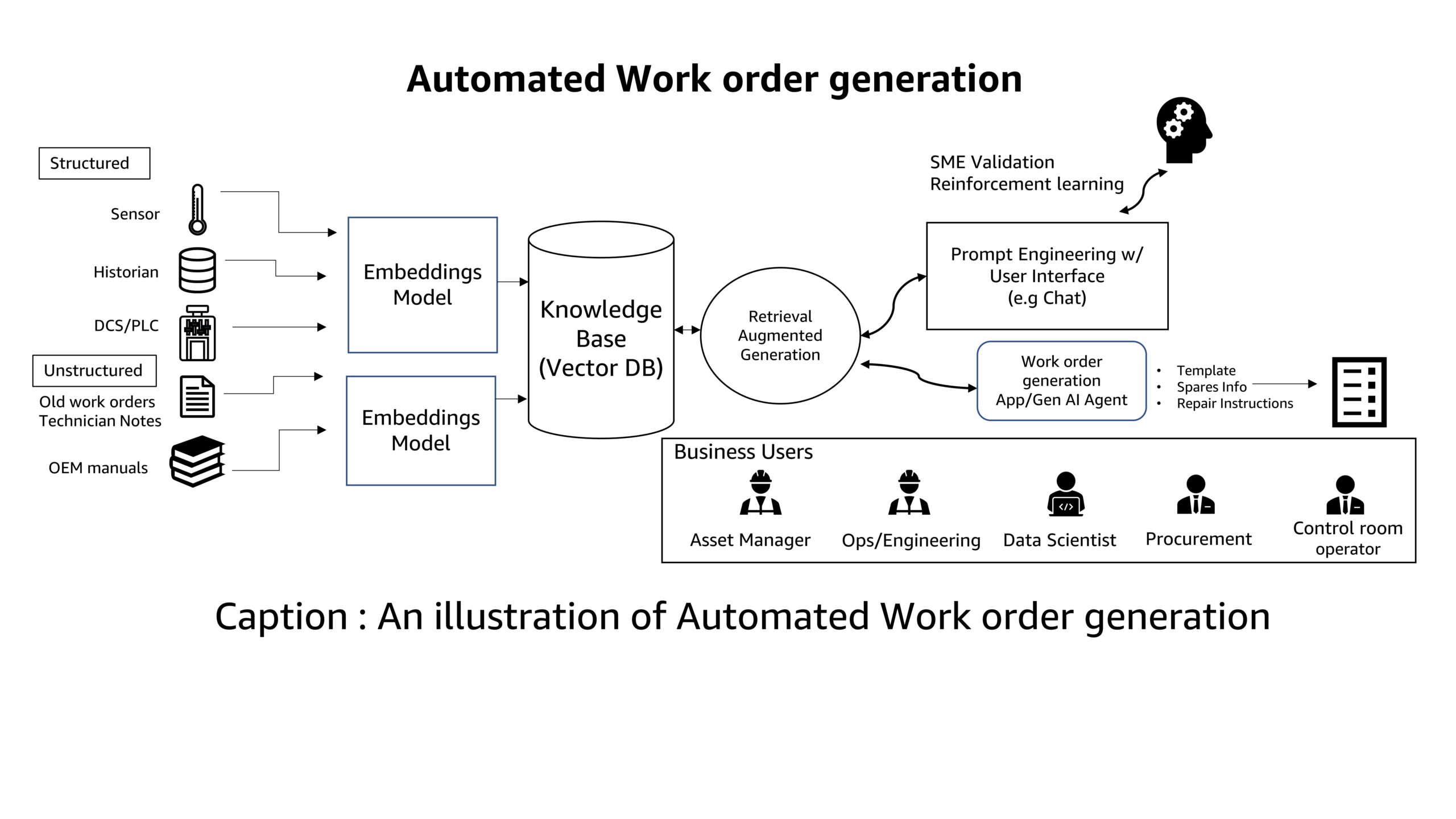
Unlike generic large language models, an expert learning language model fine-tuned for industrial maintenance leverages contextual knowledge graphs, retrieval augmented generation (RAG), agentic workflows and source relevance scoring to ensure accurate and actionable insights. By integrating structured asset data with real time sensor inputs, historical maintenance logs and process conditions, these models dynamically adapt to evolving operational patterns, refining RUL estimates and optimizing maintenance strategies with minimal human intervention.
What sets generative AI apart is its adaptability. As conditions evolve in real time from shifting usage patterns to sudden weather changes, generative models self-update to keep predictions accurate. This dynamic learning ensures maintenance strategies stay proactive and relevant as RUL estimates continuously refine, allowing smarter risk based scheduling and optimized workloads. The system reprioritizes tasks based on the latest risk assessment, preventing downtime while eliminating unnecessary servicing.
Phase 5: Automated maintenance planning, asset strategies and execution
Generative AI transforms maintenance planning into an autonomous, self-optimizing process by synthesizing structured and unstructured data, including sensor telemetry, maintenance logs and technical manuals. Unlike conventional AI, generative AI leverages RAG, multimodal learning and agentic workflows to generate context-aware maintenance strategies.
For predictive scheduling, generative AI integrates time-series anomaly detection with transformer-based sequence modeling, identifying subtle degradation trends beyond static thresholds. Reinforcement learning models simulate intervention scenarios, factoring in operational constraints, part availability and failure probabilities to dynamically adjust servicing windows.
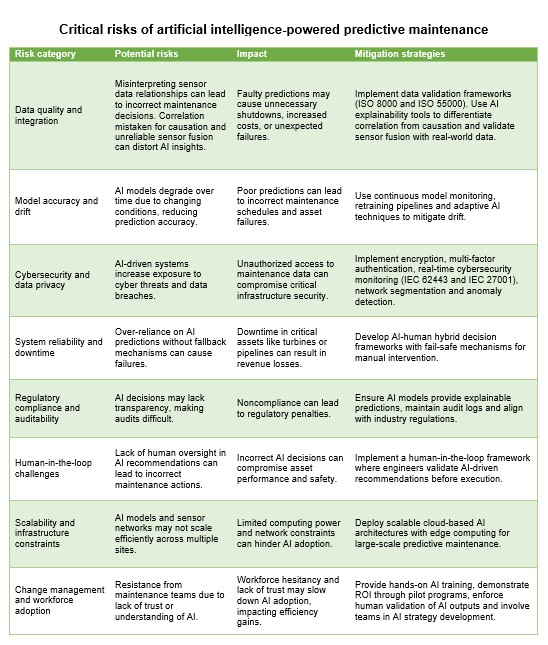
Beyond planning, generative AI-powered natural language models generate detailed work orders, extracting task-specific procedures from CMMS records and original equipment manufacturer manuals. Vector embeddings enable AI to cross-reference sensor anomalies with historical failures, ensuring precise corrective actions.
For failure recovery, causal inference models analyze past incidents to recommend proactive mitigation strategies. This AI-driven execution continuously refines maintenance workflows, reducing human intervention while ensuring reliability and efficiency at scale.
Phase 6: Continuous learning and feedback integration
A truly intelligent maintenance system does not just predict failures but continuously refines its understanding to adapt to changing operational conditions. Generative AI enables this by creating a self-learning loop where real-time feedback from past maintenance actions enhances predictive accuracy and decision-making.
Through post-maintenance analysis, generative AI evaluates predicted failure patterns against actual outcomes, refining its forecasting models. This ensures maintenance interventions become increasingly precise over time. With self-optimizing AI models, real-time sensor data, environmental changes and historical performance dynamically update failure predictions, keeping maintenance strategies relevant.
As new assets, sensors and failure mechanisms emerge, generative AI continuously integrates fresh data, retrains models and adjusts strategies. This self-improving system minimizes downtime, enhances reliability and ensures AI driven maintenance evolves alongside industrial assets for long-term efficiency and cost savings.
Enhancing reliability through collaboration
Generative AI transforms predictive maintenance by automating insights and optimizing asset reliability, but human expertise remains critical to ensure accuracy, practicality and trust. AI-driven recommendations should be validated with a human-in-the-loop approach, where engineers and technicians assess AI outputs before execution. This ensures that AI aligns with real-world operating conditions, minimizing false positives and preventing unnecessary maintenance.
Engineers refine AI recommendations by contextualizing sensor data, failure patterns and environmental variables. Human feedback continuously improves AI models, fine-tuning predictions and adapting to evolving operational demands. By combining AI’s analytical power with human oversight organizations create a reliable, intelligent and adaptive maintenance system that optimizes uptime and cost efficiency.
While generative AI enhances predictive maintenance, it introduces challenges that must be addressed for effective deployment. The AIGA Hourglass Model of AI Governance highlights the need for structured oversight, balancing AI automation with human accountability to ensure safe and explainable AI systems.
Data quality and integration remain critical, unclear sensor relationships can lead to incorrect maintenance actions. Implementing ISO 55000-aligned data governance practices, supported by IEC 61987-compliant semantic models and ISO 8000 data quality principles, helps ensure correlation is not mistaken for causation.
Model drift can degrade AI performance over time. Continuous monitoring, retraining pipelines and adaptive AI techniques help maintain accuracy.
Cybersecurity risks increase with AI-driven maintenance, expanding exposure to cyber threats. ISA/IEC 62443 and IEC 27001-compliant security measures, including encryption and real-time anomaly detection, safeguard infrastructure.
To ensure system reliability organizations must not over-rely on AI-driven decisions. Fail-safe mechanisms and human-in-the-loop frameworks should be in place, allowing engineers to validate AI-driven recommendations before execution. Regulatory compliance also requires transparency, where AI models must provide explainable predictions and maintain audit logs for accountability.
By embedding AI governance principles and aligning with industry standards organizations can deploy scalable, secure and reliable generative AI-powered predictive maintenance systems that enhance operational efficiency while maintaining compliance and safety.
The future of maintenance is AI-driven and proactive
Generative AI is transforming predictive maintenance from reactive fixes to intelligent, self-optimizing strategies. By starting small, piloting AI models on critical assets organizations can build trust, refine accuracy and gradually scale to enterprise-wide adoption.
With real-time data, AI driven diagnostics and human oversight, businesses can maximize asset reliability, reduce downtime and optimize costs, creating a future where AI powered adaptability, continuous learning and proactive decision-making drive industrial efficiency at scale.