Overcome the obstacles facing the oil & gas industry with the Internet of Things (IoT), which has had a major impact.
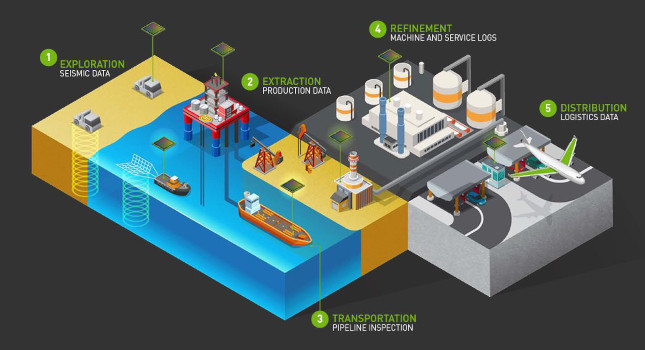
Since its introduction, the Internet of Things (IoT) has rapidly changed the oil & gas (O&G) landscape. A recent survey from Inmarsat Research revealed that nearly three quarters (74%) of O&G firms have deployed at least one IoT project, with a further 81% indicating they plan to accelerate their IoT adoption in response to challenges related to the recent global pandemic. And while these new digital technologies offer greater control, deeper insight, and more efficient operations, one of the biggest hurdles is overcoming air-gaps in remote, off-grid locations and handling the massive amounts of data generated by IoT sensors and devices.
According to some sources, a single offshore drilling rig can create over one terabyte (1TB) of data per day, especially due to recent innovations in drilling tools, such as logging while drilling (LWD) and measurement while drilling (MWD). But what value is this data driving especially if it is difficult to capture and connect to other sources?
McKinsey & Company estimates that 99% of IoT data generated in O&G is never used for decision making. At the same time this data is incurring real costs to ingest, process, and store with the hopes it will provide value in the future.
Enter artificial intelligence (AI) and machine learning (ML). AI and ML promise the ability to examine and sort through mountains of data to generate actionable insights. Data scientists have already developed a variety of ML models to optimize consumption and costs, predict equipment failures and maintenance requirements, optimize remote field operations, and improve safety. Yet we continue to see O&G enterprises up and down the supply chain struggle to operationalize these models into real world conditions.
In my experience working in IoT across multiple industries, the key to success comes not from developing models in the cloud, but rather deploying them in the field, close to the data source where the decision making needs to happen. Why?
Typically, there are three main blockers that prevent AI/ML from generating value from data at the edge 1) the distance between devices and consistent internet connectivity, 2) the inability to monitor the ongoing performance of models in real world conditions, and 3) the compute-constrained nature of the edge environment.
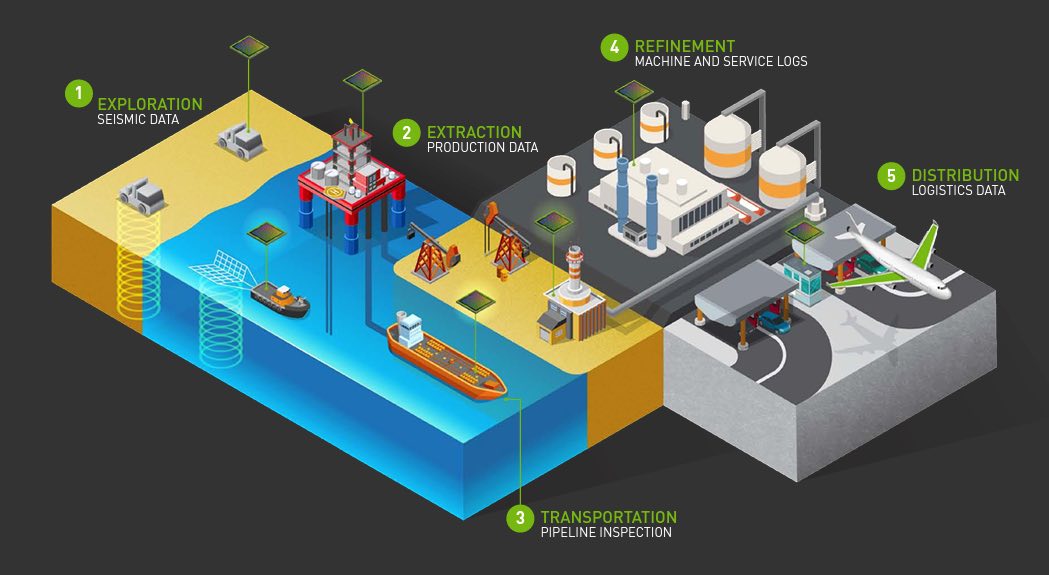
Going the distance
Sites where hydrocarbon exploration, production, transportation, and refinement happen are usually remote. Which means:
-
The location might not have an internet connection in order to deploy a model trained in the cloud or to relay sensor data back to the cloud.
-
Connectivity may not be reliable enough or have sufficient bandwidth for the
-
Even if connectivity is available, the latency (delay) from relaying data from the source to the cloud, running a model, and then returning the results back to those on the ground may be too high, particularly for control loops where latencies are measured in.
Satellite internet services offer the option of connectivity in remote locations and the next-generation LEO (Low-Earth Orbit) constellations such Starlink and OneWeb offer improved bandwidth and lower latency. However, these services are still impacted by harsh weather which can reduce uptime below that required for critical operations. The solution is local model deployments, either on-device or to a local server in the field, which provide consistent availability and latency and transmit the monitoring and observability data when connectivity permits.
Model monitoring
It’s easy for data science teams to become so focused on deploying models and running models at the edge, that they forget to think about what happens once the model is deployed. As the environment changes the conditions on which a model is trained may no longer hold.
Consider a model for predicting when a certain piece of equipment might fail based on sensor data. As the ambient temperature changes so might the significance of certain signals coming from the sensors. A model built for use in the summer will likely need to be updated for colder winter months. More broadly, ML edge operations must have the ability to monitor performance and push updated models to the fleet (or a portion of the fleet), to return observability data for continuous analysis. Observability data allows automated tools to perform continuous statistical analysis, comparing current runs to prior behavior to detect data or model drift (anomalies) and find problems before they can turn into failures.
Edge environment compute constraints
Edge devices are frequently constrained by CPU power, memory, and/or network bandwidth. Having them offload the inference data to a remote datacenter is one solution, but what happens if that introduces too much latency or requires more bandwidth than is available? ML teams need flexibility to deploy model pipelines anywhere, and everywhere, from on-device to the cloud. However, whether running on-device, on a local server, in a near-by micro-datacenter (near-prem), or in a traditional data center, specialized ML inference engines are needed to efficiently run and perform consistently across a wide range of environments to provide the monitoring capabilities data scientists depend on.
O&G is critical to the global economy. Every day companies face challenges ranging from equipment failures, leakages, safety issues, and economic penalties. IoT and AI/ML offer the promise of improved operations, but overcoming edge ML challenges is critical to any successful deployment. Operationalizing ML with dependable deployment platforms that can work in the cloud and at the edge, will help the industry generate greater value from more of their data, providing key benefits such as early detection of faults, proactive maintenance reminders, dynamic flow control, and leakage detections.